KEY CONCEPTS
•
Each stage of model complexity requires different types of simulations.
•
The human collaborations behind model integration can be as complex as the models themselves.
•
The ultimate test of a model is how well it simulates real-world conditions.
Research into roller bearing systems in electricity-powered transportation began in the 1940s, says STLE member professor Oliver Koch (RPTU University Kaiserslautern- Landau, Germany), when engineers created models of currents in the bearings of electric streetcars. This area of modeling became inactive for several decades, he says, but it has recently experienced a resurgence with the growth in demand for electric vehicles (EVs). “With all the electrification and with the new frequency converters, we get a lot of parasitic currents within our mechanical systems, and that brings us to a new level of problems.” Fortunately, today’s scientists and engineers are providing solutions to these problems using complex simulations and testing of mechanical parts interacting with electrical currents and lubricant films.
Large bearing manufacturers use modeling and simulation to meet their customers’ application requirements for light and commercial vehicle components and designs. OEMs and other customers provide them with specifications on the sizing, function and performance requirements, which serve as input into their in-house modeling software. The software has physics-based algorithms for calculating bearing loads, speeds, power loss and other information necessary to determine how well a bearing will perform under the proposed operating conditions in a specified environment. The software also contains information on the characteristics of various lubricants, which is used to calculate viscosities and film thicknesses, for use in evaluating an appropriate lubricant for the application.
From components to systems
Increasingly, models are being pushed beyond the component level, incorporating more of the interactions that characterize the ways that these components interact in a subsystem or an entire system. “What do we define as a system?” asks STLE member Daniel Merk, senior expert, advanced research and validation, for Schaeffler. “Is your system the complete set of e-axles for a vehicle, or does it also include the e-motor, inverter and gearbox?” he asks, referring to axles, motors and other components that are specific to EVs. Many companies in the industry, he says, are evolving from electronic component suppliers to full system suppliers, which requires changes to engineering operations. Component simulations might involve the rolling elements and cage of a single bearing, but systems simulations also must include all the bearings and gears in a gearbox, the electrical components with which they interact, on out to the axles, and potentially even the wheels.
At each stage of complexity, the types of simulations change. While a lubricant formulator might be most concerned with molecular interactions and atomic-scale surface properties, and a materials scientist might look at micron-sized surface textures and interfaces, a systems simulation looks at temperatures, loads, electrical currents, failure and wear mechanisms and other macroscale properties. Systems-scale models in the final simulation of a construction phase can incorporate thousands, or even millions, of possible combinations of descriptors, Merk says. These simulations build on the initial rough approximations and general characteristics arising from earlier stages of modeling, and they go on to optimize critical parameters and provide a more realistic picture.
For example, as EVs incorporate batteries with greater power densities, electrical currents in the motors affect more than just the bearings, adding to the complexity of modeling mechanical stresses, loads, speeds and temperatures. Lubricant formulations are becoming more complex, and they are operating under higher loads and increasing electrical currents. These changes are happening so rapidly, Merk says, that modelers need to optimize the descriptors of their systems in as much detail as possible in order to anticipate changes in performance that these new developments introduce.
Different types of models are required to simulate individual bearings and the more complex interactions among multiple bearings and gears in a gearbox or generator. Different models also are required at various stages, moving from the early design phase to the final optimizations. In addition to modeling the behavior of the bearings themselves and the systems of which they are a part of, outside influences like power density come into play, as well as the interactions between electrical currents, mechanical interfaces, temperature and pressure. “You need to simulate all the parameters,” Merk says.
Bearings are operating under increasingly extreme conditions, presenting a moving target for simulations development. “Progress won’t end in the near future because there isn’t a fixed stop date when we can simulate everything,” Merk says. His group is developing better simulations all the time, but the requirements for these simulations are always changing, and new models must anticipate their customers’ future requirements as much as possible. Not only that, but new simulations must be validated against real-world testing.
Detailed models, simulating multiphysics effects at the system level, would be valuable in producing more realistic representations of reality, says Fabrizio Mandrile, manager in the SKF Group’s Advanced Engineering Competence Centre. Current efforts couple conventional theory-based simulation models with new understanding and recently developed models that can capture phenomena that potentially harm the bearings. These efforts are intended to produce more accurate predictions on the component and subsystem level
(see Figure 1).
Figure 1. Thermal simulations of an electric vehicle gearbox. Figure courtesy of SKF.
In-house models often apply to research at a more fundamental level than the modeling capabilities that a company provides for its customers to use. The in-house models contain core, proprietary information, and company experts have a deep understanding of how to choose and set up an appropriate model and interpret the results. “We need to provide customers with information that actually helps them,” Mandrile says. In most cases, that involves a more applied approach to solving specific problems or evaluating specific design options.
The choice of a model also depends on the level of complexity required to provide a satisfactory answer to a given question, Koch says. “We have very high-end models in the background, and we generate simple equations that can be used for daily business,” he adds. A bearing manufacturer, for example, might need a fast, easy-to-use model to design a bearing for ordinary applications. However this simple model could be based on a much deeper foundation of knowledge that has been distilled down to the parameters that have the greatest impact on performance or the properties of interest to the manufacturer.
What’s different about EVs
The process for creating models for EVs is the same as that for internal combustion engine (ICE) axle modeling, says Chris Marks, chief engineer, on-highway, The Timken Co., but there are differences in the parameters and descriptors for the operating conditions and environment. Modeling is used mainly for the e-axle and gearbox applications, he says, but can extend the model out to the wheels if necessary. At present, he says, customers have not explicitly defined specific requirements relevant to operating in an electrical environment. There is, however, an interest in understanding what product enhancements or modifications a company can provide to mitigate the risk that electrical currents may cause, so that they can take steps to prevent premature bearing damage or loss of performance. Marks notes that much of this interest in risk mitigation stems from performance issues that customers have run into while testing and building gearboxes and bearing systems.
As interest in EV designs increases, Marks says, modelers draw on their expertise with other markets to simulate the highspeed bearings needed for electric motors and drive trains. For example, wind turbine bearings and gearboxes are very close to the electrical generator, so wind turbine models must account for stray currents and their effects on mechanical components and lubricants. “From a mechanical power transmission perspective, a gearbox is a gearbox is a gearbox, and so it’s a scaled phenomenon,” he says. “From a modeling perspective, building these systems is new to the people who have worked on conventional [vehicle] axles for a long period of time. But modeling these types of systems in general is not new. Software has been developed to handle most types of mechanical power transmission components, things that generate loads in applications and, correspondingly, impact the bearing in some way.”
In addition to putting familiar parts into unfamiliar environments, EV modeling also involves running these parts closer to their performance limits to see how they stand up. Marks notes that, for example, he consults with his in-house colleagues working on high-speed market applications in machine tools or in aerospace applications to help leverage deep application knowledge whether a tapered roller bearing, for example, must be enhanced to perform under the higher speeds in an EV.
Marks notes that electrification or e-axles bring different challenges. “Typically, many of the bearings in an e-axle are running at higher speeds, obviously, than in a conventional axle. Bearings also are generally located adjacent to a heat source, meaning the electric motor itself. And you may be using an aluminum housing, where the thermal conductivity is higher than cast iron. So, there are the factors of heat transfer and thermal expansion to consider.” High speeds and electrical currents may necessitate using different materials like ceramic rolling elements in EV bearings, he adds. The gearing also is different for EVs, Marks says. EV gearbox designs typically use helical gearing, and this type of gear design induces heavier radial loads and smaller axial loads. This is in contrast with the hypoid axles on ICE vehicles, he explains, where the gear forces acting on the bearings, and especially on the pinions, see high radial and axial loads. This difference in force distribution requires a different strategy for selecting EV bearings.
The EV industry also is coming up to speed on the best formulations for lubricants. For example, Marks says, the new lubricants might not use the same extreme pressure (EP) additives that are used for hypoid gears in ICE vehicle axles. EV lubricants must be insulating to protect against damage from stray currents, but not so insulating that static electricity builds up, which could cause arcing. Because of the high speeds in an EV motor, foaming in the gearbox can be a concern. Often, EV lubricants also serve as coolants, and this dual function could result in the bearings running slightly warmer than they would in a similar application in an ICE engine. “There’s a lot of things to consider,” he says. “And the environment is different than the conventional space in many ways.”
Merk agrees—EV motors have different lubricant requirements than for ICE vehicle lubricants. For example, lubricants in contact with the electronic components of an EV must protect against copper corrosion. Electrical currents can change the characteristics of oils and greases. With the development of new lubricants and additives, new mechanisms come into play. Lubricants, in turn, must perform new tasks as EV motors evolve. Lubricants that serve as both lubricant and coolant, for example, must be compatible with a variety of mechanical components and interact with electrical currents in a specific way. A change in the material or design of one component can affect the flow of electrical current in the system, and the current flow can affect the aging of the oils and greases through which it flows. Each new motor design changes this interplay, requiring the corresponding simulations to be re-optimized. Merk notes that this effort requires a continual interaction between human and artificial intelligence (AI), to speed up the processes that can be automated, while ensuring that the simulations accurately represent the actual vehicles on the road.
The challenges of modeling EVs also are distinct from those typical of electrical motors applied in traditional industrial operations, says Mandrile. Electrically induced bearing damage (wear caused by electric discharge), noise and vibration and other factors are of greater concern in EVs. Because electric motors are much quieter than those of ICE vehicles, drivers and passengers are more aware of any sounds and vibrations coming from the EV.
Traditional elastohydrodynamic lubrication (EHL) calculations and the electrical discharge model in bearings are completely separated phenomena, says Mandrile. In real life, however, if an electrical discharge causes a temporary lubricant film failure, the result is brief metal-to-metal contacts that damage the bearing’s raceways as well as negatively affecting the local material fatigue properties of the surface. A new combined model is needed, he says, to predict the disruption to the EHL behavior by the constantly changing raceway topography and the local weakening of surface properties.
Building the models: Physical foundations
STLE member Bill Hannon, manager – product R&D, The Timken Co., explains that a common modeling approach starts with first-principles physics, validated using laboratory testing. “The key to testing methodology mimics modeling; we want to predict one small event and validate it, and then grow that to a system level knowledge,” he explains. This multifaceted modeling effort requires an equally multifaceted team to build and validate the models, he says. “And it’s a challenge to get everyone to talk to each other. But that’s also part of the pleasure, right? We’re here to solve a puzzle; we’re here to undo a riddle.”
Building a systems model involves more than simply adding separate components together. For example, Koch says, some models for calculating the electric capacitance of a bearing calculate the resistance and capacitance of each contact between the rolling elements and the outer ring, and they add these together to build up a network of contacts. However, this approach can yield a total capacitance value for the bearing that is off from the measured value by a factor of 50, he says. “So we have to understand what’s going on, and that means we really model the whole bearing with different tools.” To validate the model, they make bearings in which every contact but one is insulating, using ceramic components or coatings, and run lab tests to measure each conductive contact in isolation. “We took a bearing and isolated everything except the contact between the cage and the rolling element, for instance, to see if our model was really doing this particular contact in the right way.”
Creating and applying a model effectively requires knowing up front what the customer is looking for and choosing the most relevant parameters for the simulation. “What are you interested in? What are your verification criteria?” asks Mandrile. Making sense of the output also requires a knowledge of what constitutes a physically reasonable result for the component or system under consideration.
Multidisciplinary, multiscale, multiphysics models
One of the main challenges to this type of research, Koch says, is bringing together people with different skills and different education: mechanical engineers, electrical engineers, chemists and modeling specialists and getting them to understand each other’s terminology and methods. Setting up collaborations is not trivial, Koch says. In addition to differences in terminology, modelers in different specialties tend to focus on different things.
Some mechanical engineers, for example, investigate situations that their industrial counterparts face with respect to stresses, failure and damage modes and other aspects affecting mechanical parts operating at the micron- to macroscale under high temperatures and pressures. Meanwhile, chemical models of lubricant systems often focus on homogeneous, short-molecule synthetic fluids because of the simulation time and effort involved, unlike the models of complex molecules and reaction systems often found in other areas of chemistry. Koch uses simplified lubricant models for fully formulated oils or greases to calculate film thicknesses and other basic properties.
Electrical engineers are accustomed to modeling currents flowing at ambient pressure between polished surfaces separated by millimeter-scale interfaces, Koch says. The gigapascal pressures, surface asperities and micron-scale contact regions that are familiar to mechanical engineers (not to mention complications arising from lubricant oils and additives) often present unfamiliar challenges to researchers who develop the algorithms underlying models of electrical current flow and spark formation.
Bearings pose particular challenges to modeling and simulation efforts, not the least of which is the wide variety of bearing types and materials, says Mandrile. Bearings exhibit nonlinear behavior, and their interaction with other components can have significant, difficult-to-predict effects on the entire system. Bearing functioning and failure occurs on a wide range of scales, from the microscopic to the macroscopic, and the details of a bearing’s materials and design can be proprietary knowledge.
1 Designing or applying models without this knowledge can involve a great deal of trial and error to produce results that replicate lab tests.
In an effort to overcome these challenges, the German Collaborative Research Association for Drive Train Technology (FVA GmBH), a consortium of 200 companies, is doing precompetitive research. “One of the big topics nowadays is electric currents,” Koch explains. “This topic has a very high response in industry, so industry is giving the question to the universities, and universities like my institute are working on the topics, together with electrical engineers. And we are presenting the results to the industry.” He adds that the working group dealing with electric currents in mechanical systems
(see Figure 2) is one of the largest groups, drawing interest from lubricant and gear manufacturers, automotive suppliers, OEMs and other large companies. “And of course, there also are the bigger companies developing their own methods, and they compare to see if we get the same results as what they develop, and they use our data for more validation and so on.”
Figure 2. FVA GmBH, a consortium of 200 companies, uses several modeling approaches to investigate the role of electrical currents in mechanical systems: a.) schematic of a bearing, showing a segment used for the models; b.) finite element modeling (FEM) simulation for a lubricated conductive rolling element in an electric field; and c.) elastohydrodynamic lubrication (EHL) simulation for a lubricant film in an electric field. Figure courtesy of professor Dr. -Ing. Bernd Sauer and professor Dr. -Ing. Oliver Koch.
Does the model represent reality?
Computational models are based on established physical principles and validated with laboratory testing. AI also is used to facilitate the usage of complex physical models, making them accessible to designers and application engineers in their design tasks. “Nothing is invented; it’s not magic,” Mandrile says. Other fields (biomedicine and materials discovery, for example) may use unguided AI to scan a parameter space for new compounds or to search for patterns in large databases. However, designing mechanical parts for real-world applications—even with the support of supervised and physics-informed machine learning—still requires human analysis and evaluation of the modeling results to ensure that they provide realistic predictions of the real-world systems they are simulating. “Without testing or without validation, you’re just basically guessing,” he adds.
As specific parts of the model become more established, less validation is required for these parts. “You can’t test everything every time,” says Mandrile, but you can build confidence in the software. Less testing of the established parts of a model allows engineers to focus on validating models for new situations, components or systems. He notes that some OEMs use “virtual validation,” testing their applications with digital twins of their systems. This doesn’t replace real testing yet, he adds, although it already reduces that need.
For some phenomena, a theoretical model doesn’t even exist. In these cases, fundamental testing is required as the basis for a new model. “We are constantly working to improve our understanding of reality to improve and expand our modeling capacity,” Mandrile says. “But the first step is always to visualize, understand a phenomenon on maybe a fundamental testing level, and then transform that into a model that describes the onset and evolution of that phenomenon. And that eventually will lead to a more powerful simulation tool, one that can operate and predict a wider range of effects.”
In the laboratory, model validation efforts involve a range of test rigs that go from the individual component level to the system level. “Every application has its unique flavor,” Hannon says. The emphasis might be on duration or noise levels, or it could be on high operating speeds, thermal response or performance under heavy stress. “We don’t just do finite element analysis,” he continues. “We’re calculating the flow of oil; we’re calculating how you build a fluid film. We’re calculating the high-pressure viscosity of the lubricant. Then there’s mixed mode friction that generates more heat, and that heat thins the oil. And so now the oil film thickness is different.”
“When we begin validating, our first thing for the lubricants is that we want to quantify the lubricant by its rheological performance,” Hannon says. “And once I understand how the lubricant’s working, then I’m going to up my game—can I predict the performance of a ball on a plate? Once I get to that level, where I’m predicting the performance of an individual component, I now want to say, ‘Can I do it for many components?’ and now I want to study the bearing itself. So, we grow our comprehension, and by the time we insert it into the entire system, what we’re doing is we’re validating everything from elasticity— do I have the right load zone—all the way out to the bank performance saying, ‘Do I have the right heat source? And therefore, the right heat sink? Can I get the right temperatures? Can I get the right power loss?’”
“There are lots of test rigs,” Hannon says. “There are big test rigs, little test rigs and even test rigs that test individual components. There’s a tribological lab that studies tribology tests of one-off ball on a plate or cylinder on a disc. Or it could just be one standalone bearing that you really want to learn about. And then there are the next level of test rigs where you go small bearings, running the duration, do I have the proper fatigue performance, and then you can bring it up another level. And you can say, now I’m traveling at a high load and a high speed. And as I changed through my load cycle, how does the bearing perform, and how is its sound behavior?” Although the physical principles remain the same and a product design team can borrow concepts and strategies from a company’s other product lines, if the model doesn’t agree with the lab validation results, the model must be adapted to better fit the real-world observations.
Modeling isn’t just for finding performance limits; exploring the parameter space also can yield new insights and predictions that help customers optimize their system designs. Marks says, “It’s not always just us, giving them an answer for hey, this bearing should last x, right?” The automated bearing design algorithms enable them to characterize complicated duty cycles in far less time than laboratory testing would require. In that way, modeling and analysis are major contributors to the efficiency and productivity of an engineering team.
“Modeling software does have capability to run things like Monte Carlo type simulations, which can be very beneficial to an engineer, if they’re trying to study the effects of various parameters,” Marks says. He cites the example of a customer looking at the interaction of different shafts in their gearbox and the relative angle of those shafts to each other. Monte Carlo analysis produced an optimum shaft geometry that allowed the customer to use the same bearings that they had selected, but they improved the performance of the gearbox by arranging the shafts to optimize the shaft loads in the system.
“The value is that the models have been validated against actual experimentation—all the different parts and pieces, the physics,” Marks says. “Where we leverage it is from a product development standpoint. For customers, especially in EV, speed to market is very important. And they’re relying more heavily on analytical models to minimize physical testing time and cost. It’s really confidence in the tools we have, and then customers having confidence in us and working with us. It’s not so much that we do not perform testing—it’s more so that we try to do more analytical work, so we have to do less testing or less iteration in the product development process.”
One challenge to validating models is a lack of standards for tests of the electric properties of lubricants, Koch says. The German Standardization Institute has formed a committee to come up with standard test methods. His colleagues have developed the GESA test rig
(see Figure 3) to measure electrical properties of lubricants, and this rig will serve as the basis for standardization in the future, he says. Several companies are currently conducting round robin tests on their own copies of the GESA test rig, and standardized procedures are being developed.
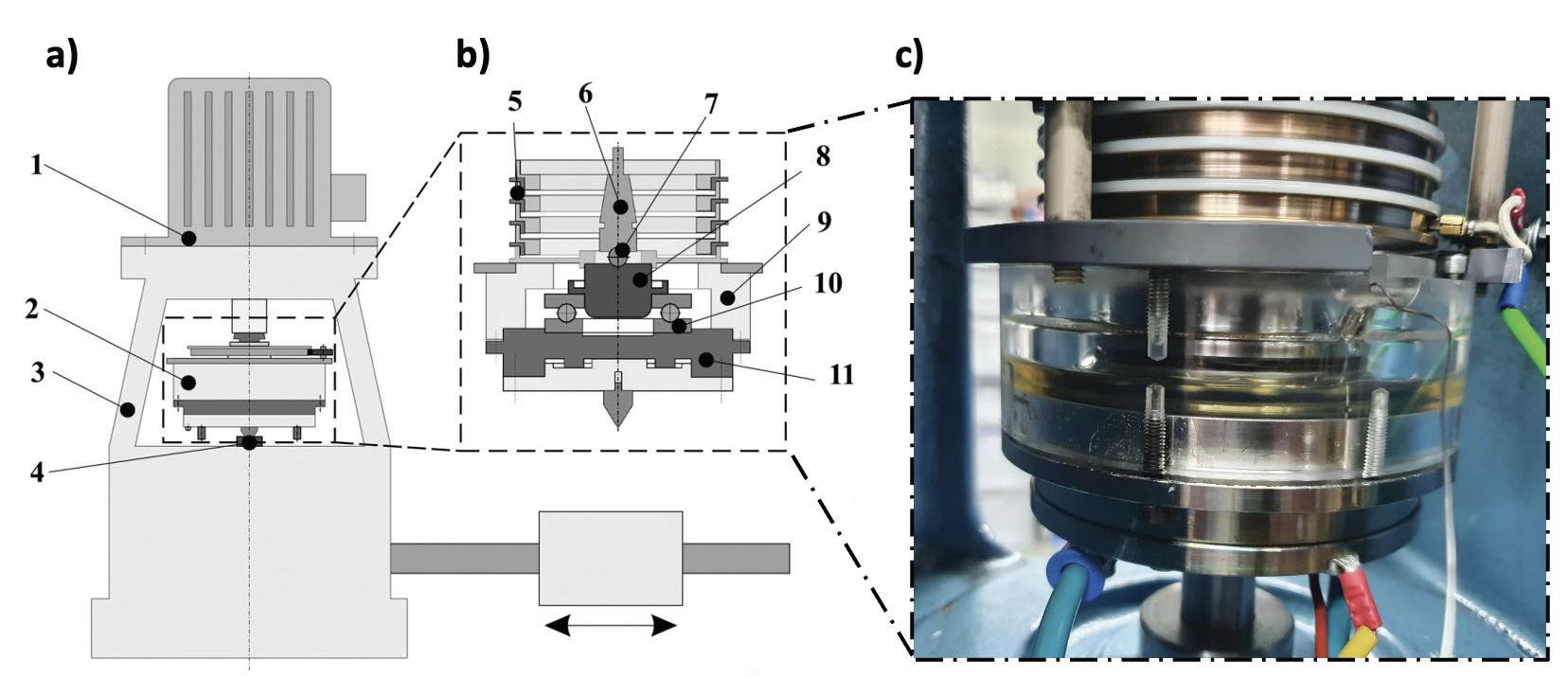
Figure 3. GESA is a functional module device for extended lubricant analysis: a.) GESA mounted in a four-ball testing apparatus. 1: driving motor; 2: GESA; 3: housing; 4: axial load punch. b.) Cross-sectional view of GESA. 5: distributing ring; 6: drive shaft; 7: centering ball; 8: shaft; 9: housing; 10: tested bearing; 11: bearing ring holder. c.) Photograph of GESA. Figure courtesy of professor Dr. -Ing. Bernd Sauer and professor Dr. -Ing. Oliver Koch.
Standardized tests will be critical to constructing whole-system models, Koch says, so that lubricant models can be integrated with bearing and gear models. He notes that he plans to evaluate the abilities of a test rig to provide input to such whole-system models. He is in contact with STLE Past President professor Ali Erdemir, whose research group at Texas A&M University is investigating chemical interactions between lubricants and solid surfaces at the contact level. Koch emphasizes that these types of collaborations are necessary to provide the different areas of expertise necessary to build additional capabilities into their models. “What kind of question do we want to answer? And then you have to choose the right level of modeling,” he says, referring to the different approaches needed for molecular modeling, component-level modeling and systems modeling.
In addition to standard tests, standard nomenclature and definitions are needed to ensure that data and results can be compared among different models. Toward this end, the German Research Institute for Drive Technology is developing the Reusable Engineering Exchange Standard (REXS), an industrywide standard for uniform modeling nomenclature and definitions for gear units and components, to facilitate data exchange for gear unit development.
2
Automation and optimization
Most of these simulations can be run on typical office computers, Koch says, although some of their finite element analyses must be run on the university’s computing cluster. Koch notes that typically, the longest of these sorts of simulations can be completed overnight. So far, they have not used automation to a large extent because as academic researchers, they focus more on generating knowledge and gaining a deep understanding of what the simulations are telling them about the fundamental phenomena in their systems.
Automation can be more useful in an industrial setting, where the same test must be run multiple times, Koch says. For example, a bearing designer might evaluate a specific change in a rolling element or cage to see whether the electrical load in the bearing rises above a critical value. A series of simulations over a certain range can establish optimum values for specific parameters.
Model automation also is emerging as a way to reduce engineers’ time and effort by taking over repetitive tasks such as data search and retrieval, data formatting, routine calculations, parameter optimizations and standard report generation. Automation also allows the algorithm to work on large datasets and large numbers of interacting parameters that would be impossible for an engineer to tackle in a reasonable amount of time. This allows engineers to devote more time to value-added activities.
For example, says Mandrile, bearing-specific load reduction calculations that used to take a week of work with manual inputs now take less than a day when most of the process is automated. “The routine that is running this load reduction is as automated as possible,” he says, “but the next step is building an AI algorithm into that.” The aim of building in AI capabilities, he says, is not to eliminate human intervention, but to make sure that the outputs are user-independent. By standardizing the procedure, experts hope to produce consistent outcomes, regardless of who oversees the process. Mandrile foresees that this type of standardized modeling could one day be provided as a service to internal, maybe even external, users of simulation tools.
The degree to which a model can be automated depends not only on the repetitive nature of specific tasks and the skill level of the people using the model but also on the return on the investment required to implement the automation. An operation that is rarely performed won’t be a priority for automation, Mandrile says.
Whether the modeling is done by the supplier or by the customer, interpreting the results still requires an understanding of the strengths and limitations of the specific modeling methodology involved. The user also must understand how to use the modeling output in a way that is relevant to their application. A well-developed model includes built-in quality checks to ensure that results are realistic, and that errors, error patterns and outliers do not invalidate the results.
Putting the knowledge to work
Despite the challenges, Merk says, modeling is still faster and less expensive than testing everything in the lab. Modeling allows engineers to vary and examine the effects of combining and varying large numbers of parameters, while reducing the time, resources (e.g., lubricants, electrical power, hydraulic fluid, personnel) and material waste involved in extensive lab testing.
Machine learning plays a crucial role in exploring parameter spaces, working hand in hand with human analysis and evaluation, Merk says. Smart data management practices, in which electronic data, stored in standard formats, are available to both human analysts and modeling programs, enable real-time comparisons of physical tests with parameter studies generated by the simulations. “The combination of physical tests and simulation is, from my point of view, the key benefit we have, because we know about this smart combination and the important role of that,” he adds.
For example, Merk says, lubricants can be characterized in great detail, and this information can be combined with a detailed description of a roller bearing and a theoretical simulation. The result is a multifaceted representation of a complicated mechanism that can be used to generate predictions of performance characteristics, failure mechanisms and other critical pieces of information.
Simulations go in phases, Merk says. Rough approximations are progressively refined to provide more realistic representations. The results of these simulations can guide the selection of lab tests to perform and identify the factors that contribute the most to performance (or failure). The results of the lab tests can be integrated back into the model. Eventually some aspects of the system are so well characterized that further lab testing is not necessary, which allows developers to focus their time and resources on testing new or less-understood aspects of the system.
Models can provide understanding of phenomena that are difficult to observe in the lab, Koch says. For example, he says, “Nobody really knows what electric currents are going through the mechanical system.” Bearing or gearbox manufacturers build their components according to a set of specifications, but these specifications often contain no information on electrical currents. In some cases, a specification will list a maximum current level, but it’s difficult to rely on this because there is a lack of specific information on what is happening in the system. “We have to come to the point that we are able to simulate the whole mechanical system also as an electrical model, and to see how the electric current is flowing through the mechanical system.”
Such a model would show the critical points in a system. For example, a steel bearing that is especially susceptible to damage by electrical currents could be replaced with a hybrid bearing or a ceramic bearing. However, Koch says, the question is, “What happens next?” Replacing a conductive bearing with an insulating one will cause the current to flow along another pathway, possibly damaging another bearing or gear
(see Figure 4). Thus, an effective simulation has to optimize the entire system.
Figure 4. Understanding the role of electrical currents in an e-motor requires looking at interactions between all of the mechanical components, lubricants and parasitic currents in the system. Figure courtesy of professor Dr. -Ing. Bernd Sauer and professor Dr. -Ing. Oliver Koch.
Premature bearing damage can be caused by transient changes in speed, torque or load direction reversals that might not appear in steady-state calculations. Mandrile cites the example of electrically assisted farm tractors, whose transmissions experience frequent, strong speed transients and motion reversals. At each zero-motion point, the lubricant film in the bearings also can go to zero, potentially causing surface damage. Kinetic energy recovery systems, like the regenerative brakes on some cars, motorcycles and even bicycles, also experience frequent torque or speed reversals, and models must reflect these transients and reversals to produce an accurate simulation.
Communication between experts in various fields, software experts and the customers is critical to the success of this effort, Mandrile says. Customers might not need the fine details of how a model was developed and applied, but they need an understanding of what the models are telling them to make decisions, and they need to know the key points from a modeling run and how to apply this knowledge to their situation
(see Engineers Take the Wheel).
Engineers take the wheel
Providing customers with more doit- yourself modeling capabilities supports a larger industry trend.
1 Engineers no longer use modeling only for late-stage verification—they are introducing modeling and simulation earlier in their development process to help in decision making and in guiding the direction of the process. This shift has provided critical early insights into designs and potential failure modes, helping engineers to avoid costly mistakes and reduce the time needed to arrive at an optimal design, thus reducing time to market.
Several manufacturing companies are providing software that lets users incorporate specific product specifications (bearing stiffness, for example) into their calculations without leaving their simulation environment
(see Figure 5). Because the models are based on characteristics of real products (while protecting proprietary information like a bearing’s internal geometry), a customer’s engineers can evaluate these virtual components directly in their models, rather than coming up with specifications on their own.
Figure 5. An engineer constructs a model of a mechanical system, using accurate stiffness data for real bearing products embedded into the simulation environment. Figure courtesy of SKF.
Other component and systems manufacturers take this concept even further, allowing their customers’ engineers to model a complete gearbox, for example, and view the components in 3D to evaluate power flow and pressure distribution.
3,4 Automated parameter adjustments allow engineers to evaluate the influence of specific parameters on a system’s performance, and combinations of parameters can be optimized for specific functions.
Systems of modelers make models of systems
Hannon notes, “Clients want to know what’s under the hood. They want to know who’s got this algorithm, and why is the algorithm over there just a little bit different? And what is the right course for my application? There are a lot of technical questions from the customer, questions that have never been brough up before. We welcome that, too.”
Marks agrees—as challenging as the EV market can be, it’s also a great opportunity to tackle a variety of new concepts and designs. Because ICE engines are a mature technology, axles, transmissions, engines and wheels look very similar from one model to the next. “They’ve been tweaked and reengineered, and the waste has been designed out over decades. But then the e-axle electrification is sort of a green space. And the architectures vary widely. There’s a lot of creativity,” he says. Although there are constraints related to space, weight, cost and safety, there is a lot of freedom to innovate. “It’s refreshing from the standpoint of a change in the industry and being an engineer getting to work on products and these applications.”
REFERENCES
1.
Ansys Blog (Jan. 30, 2023), “Bearing calculations no longer a lot to bear with easy-to-use automation tool,” click
here.
2.
FVA e.V., the German Research Institute for Drive Technology, “REXS (Reusable Engineering Exchange Standard),” click
here.
3.
Schaeffler Technologies AG & Co. KG, “Bearinx high-level bearing design,” click
here.
4.
Schaeffler Technologies AG & Co. KG, “Bearinx calculation modules,” click
here.