Progress in tribological molecular simulations
Dr. Nancy McGuire, Contributing Editor | TLT Cover Story June 2021
Computational modeling and simulation continue to make inroads into tribological systems and processes, providing a molecule’s-eye view of the action as it happens.
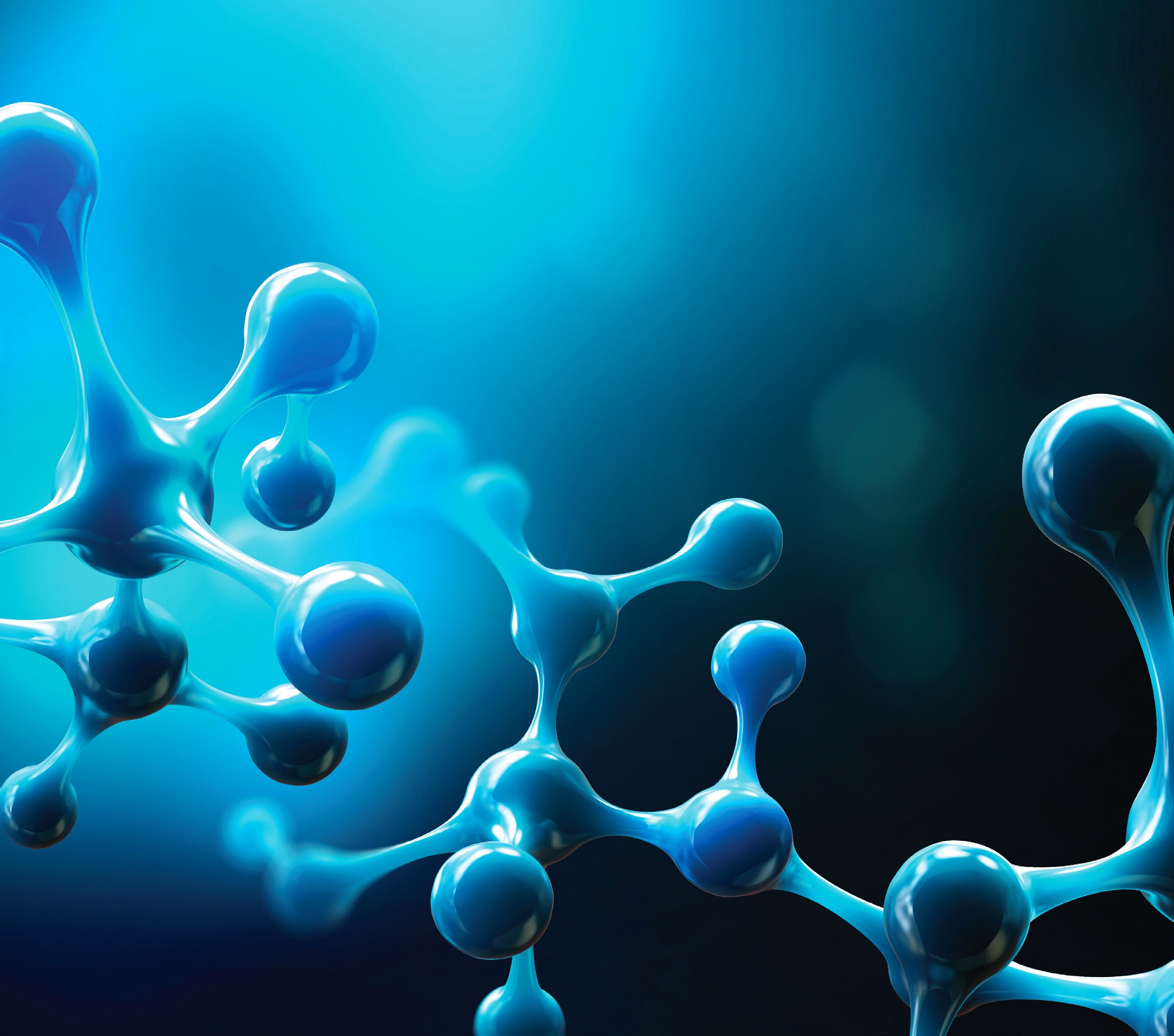
KEY CONCEPTS
•
Modeling and simulation methods work best on the time and length scales for which they were developed.
•
Advances in computing power and models enable simulations of larger systems and more complex interactions.
•
Artificial intelligence—including machine learning—could open up a host of new capabilities for tribological simulations.
In the computational modeling region between quantum physics and macroscale engineering lies the chemistry-intensive realm of molecular modeling. Not too long ago, molecular modeling of tribological systems was considered interesting basic science—but not nearly well developed enough to be of use in practical applications such as formulating lubricants, coatings and surface treatments. Things are changing now.
Computers are becoming faster and more powerful—computations that used to require a room-sized supercomputer can now be done on a small cluster or even, in some cases, a desktop computer. Today’s computers are beginning to take on groups of atoms that are large enough, and time spans that are long enough, to simulate realistic processes. Models of the physical systems underlying these processes also are evolving to include more complicated materials and interactions. And artificial intelligence could eventually allow tribologists to tackle problems on a whole new level.
Where molecules call the shots
STLE member Ashlie Martini, professor of mechanical engineering at the University of California, Merced, notes that molecular dynamics-type computational simulations are most useful where atomic or molecular scale phenomena play an important role in macroscale processes.
For example, viscosity index (VI) improvers rely on the interactions among long-chain polymer molecules, making them ideal candidates for molecular modeling. These polymers consist mainly of carbon, hydrogen and sometimes oxygen, all elements with well-characterized interatomic interaction potentials. Their interactions can’t be subsumed into continuum-based approximations, Martini says. “It’s not something that you can necessarily describe using only bulk fluid properties. If you want to understand how the viscosity modifiers are doing their job, you have to look at the molecule itself.”
At present, high molecular-weight VI improvers can put real lubricant systems far beyond the total molecular weight limit that molecular models can currently handle, Martini says. Nevertheless, model systems can provide good indications of how the VI improvers themselves behave under shear, temperature changes and other real-world conditions, she says.
STLE member Judith A. Harrison, professor of chemistry at the U.S. Naval Academy in Annapolis, Md., notes that efforts to design low-friction coatings and lubricants, starting from basic physical and chemical principles, have been hampered historically by the lack of a thorough understanding of the atomic-scale mechanisms that govern friction. Much of her group’s recent research involves modeling the interactions between atomic force microscopy (AFM) tips and solid surfaces, which lend themselves well to molecular modeling because of the nanoscale contact areas involved (
see Figure 1).
Figure 1. (Left) Transmission electron microscope image of a DLC atomic force microscope tip indenting a diamond (111) surface with some hydrogen termination. (Right) Molecular dynamics (MD) simulations of this indentation (top) at the maximum applied load and (bottom) as the DLC tip is pulling back from the diamond surface. Red represents compressive stress, and blue represents tensile stress.4
Harrison notes that contact between an AFM tip and a surface always involves some amount of sliding, intentional or not. Adsorbed or chemically bonded hydrogen on either or both surfaces, applied load, adhesion, roughness, sliding speed and sliding distances all affect friction. Her group has studied diamond-like carbon (DLC), silicon and silicon carbide tips sliding against diamond and, quite recently, silicon tips sliding against silicon substrates. Their simulations are geared toward explaining experimental measurements, while identifying underlying mechanisms responsible for the observations and elucidating links between variables that might be hard to ascertain experimentally.
Modeling is especially good for isolating the effects of variables in a system in ways that are sometimes not physically possible in an experimental setting. “That’s one advantage of simulations,” Harrison says. For example, simulations can examine just the indentation motion of an AFM tip, with no lateral motion. The precise number and locations of the hydrogen atoms on the AFM tip and a simulated solid surface can be specified and held constant, while the load or sliding direction is varied, and then these variables can be held constant while the amount of hydrogen is varied. “In this way, you can look at all the dependencies,” she explains.
A matter of distance and time
“The way that simulations work is they’re based on models that describe how the atoms interact,” Martini says. Two things go into a simulation. First is the initial structure—the positions of the atoms, corresponding to the material or chemical system of interest—based on crystal structure data, spectroscopy or other standard laboratory techniques. Second is a set of force fields (also called potentials) that uses a set of empirical parameters, such as force constants, to describe the interactions between the atoms. Simple interaction models use just a few parameters, but the more complicated models needed for modeling tribology can involve hundreds of parameters. The interaction models themselves act as approximations in the simulation, so getting the models right is key to a realistic simulation, she adds.
Tribological phenomena often push the limits of molecular modeling capabilities because of differences in the scales they address, says STLE member Hitoshi Washizu, professor of simulation studies at the University of Hyogo in Kobe, Japan. Real-life tribology involves molecules interacting on a scale of microns to meters over time periods that can span milliseconds to years. In contrast, molecular modeling typically spans length scales up to tens of nanometers and time scales on the order of nanoseconds (
see A Model for Every Scale).
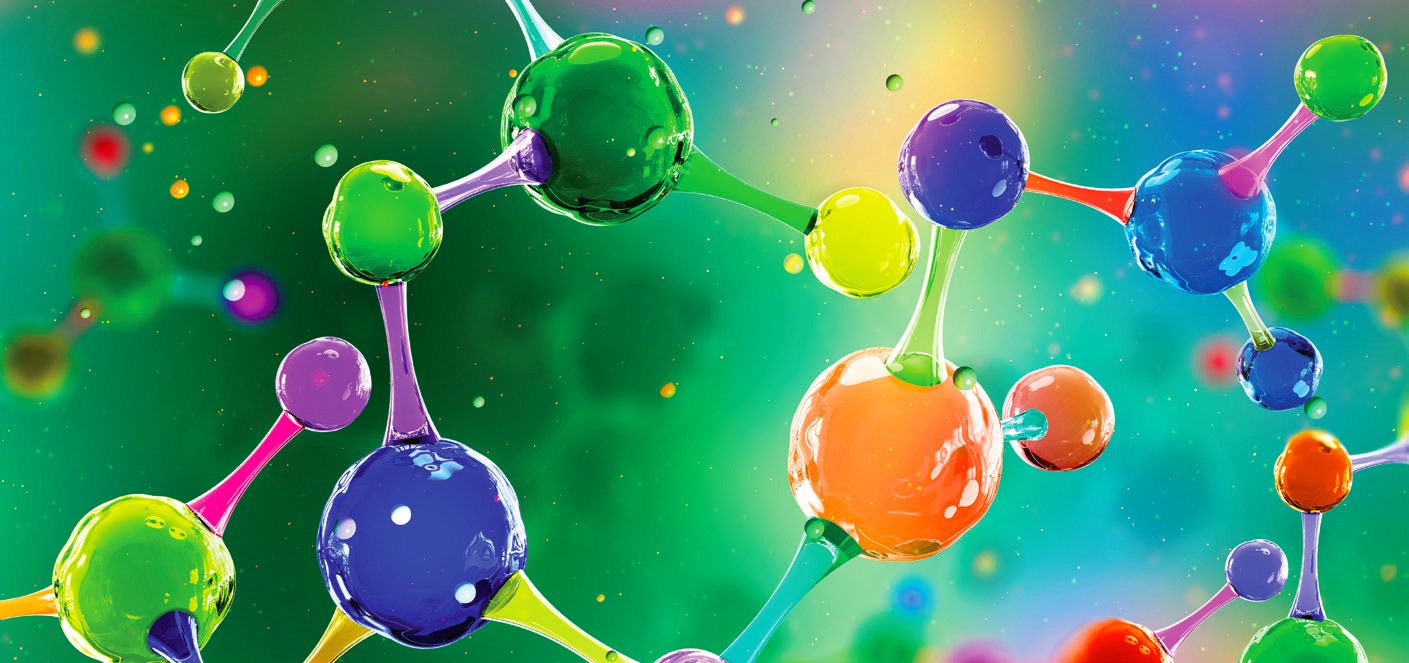
A model for every scale
Particle simulations, and the models on which these simulations are built, cover a variety of length and time scales, each suited to different types of phenomena:
•
At the quantum level (Ångstroms and nanoseconds), molecular orbital theory, density functional theory and tight-binding methods describe interactions within small clusters of atoms.
•
At the nanometer scale, reactive force field (ReaxFF) molecular dynamics and reactive empirical bond order (REBO) models use classical mechanics to describe molecular-scale interactions like chemical reactions, the formation of tribofilms and superlubricity occurring as a result of friction-induced chemical reactions.
•
Molecular dynamics models extend into the micrometer and microsecond ranges, covering larger molecular structures like elastohydrodynamic lubrication layers.
•
Langevin dynamics and the lattice Boltzmann method cover fluid dynamics processes, like the effects of shearing on VI improvers, on the micrometer to millimeter scale, over spans of microseconds.
•
Mesoparticle simulators, covering microscale processes, are used to study wear mechanisms involving two solid surfaces. Discrete element method simulations are well suited for large numbers of millimeter-scale particles and complex geometries.
•
Continuum models like finite element analysis simulate the behavior of entire mechanical components over time periods that can be replicated in lab or field settings.
Basic properties like shear rates are very different when viewed atomistically and from a macroscale (or even microscale) perspective, says Washizu. For example, at the macroscale, atomic vibrations and fluctuations are too small to consider. At the nanoscale, atomic fluctuations, diffusion, dislocations and other perturbations dominate. However, this does not mean that molecular simulations are not relevant to macroscale processes. Washizu notes that lubricant additives, for example, “swim” in the base oil, exhibiting liquid-like properties until they adsorb onto a solid surface, forming a solid-like tribofilm. Molecular modeling is uniquely suited to this type of simulation, which is not accessible using quantum mechanics or finite element analysis.
Evolution of mesoscale features like grain boundaries and dislocations (
see Figure 2) are of great importance to understanding wear, but the capabilities needed to simulate them are still in an early stage, says STLE member Izabela Szlufarska, professor of materials science and engineering at the University of Wisconsin-Madison. The effects these processes cause (e.g., hardening or cracking) can determine whether a mechanical component fails prematurely, but the processes occur at length and time scales that are inaccessible to quantum chemical modeling and only barely accessible to classical molecular dynamics techniques.
Figure 2. MD simulations of sliding of a single asperity AFM tip over nanocrystalline aluminum. (Top row) Pure aluminum shows grain boundary migration (dashed line), which eventually leads to grain growth in the tribolayer. (Bottom row) Doping the aluminum with zirconium suppresses grain boundary migration and grain growth. Figure courtesy of Yeqi Shi and Izabela Szlufarska.
However, as molecular dynamics methods and computing power continue to evolve, it becomes possible to study larger groups of atoms, says Momoji Kubo, director and professor in the Center for Computational Materials Science at the Institute for Materials Research, Tohoku University in Sendai, Japan. One recent simulation of stress corrosion cracking of polycrystalline titanium at Kubo’s lab involved 1 billion atoms, and other simulations have included hundreds of millions of atoms. At this “superlarge” model scale, Kubo says, modelers can predict more realistic material properties and optimize materials and friction conditions because factors such as grain boundaries, surface roughness, additives, textures, environments, side reactions and tribofilm generation can be included (
see Figure 3).
Figure 3. “Superlarge” models, involving hundreds of millions of atoms, can be used to study realistic material properties like friction and wear. Figure courtesy of Momoji Kubo.
Kubo works on multiphysics models that simulate interactions among chemical reactions, friction, impact, stress, electrical fields, fluid dynamics and processes that generate heat and photons. He notes that the MASAMUNE-IMR (
MAterials science
Supercomputing system for
Advanced
MUlti-scale simulations towards
NExt-generation -
Institute for
Materials
Research) supercomputer at Tohoku University, launched in 2018, has made it possible to simulate phenomena like surface roughness and wear, in addition to friction. In some cases, the results of these simulations can be validated using laboratory studies: microscopy is ideal for tracking texture and grain boundary evolution, for example.
The ability to model longer time periods is a more difficult challenge than the ability to model larger numbers of atoms, Kubo says. At present, molecular dynamics computations can extend to about a microsecond at most, and nanosecond durations are more typical. This limits the ability to model long-term phenomena like fracture and fatigue, which can take place over months to years.
Another problem with the small time steps and limited total time scales typical of molecular dynamics simulations is that it limits the ability to model infrequent events, says Harrison. For AFM tip simulations, “If you slide longer, you see more of the surface, and you have more of a chance for infrequent events to happen,” she explains. A few methods have been developed to model longer time scales and infrequent events, but they do not yet enjoy widespread use, she says.
Laboratory scientists often use accelerated processes to simulate wear, corrosion and other phenomena that occur over months or years in the field, Szlufarska says. Likewise, molecular simulations require special approaches to access the time scales of real-world processes. One approach is to perform simulations (e.g., for sliding velocities) on different time scales and use physical intuition and theory to determine how this understanding applies to experimental time scales. Another approach is to accelerate simulations. For example, increasing the temperature or partial pressure of gas in a simulation speeds up the simulated chemical reactions. Ensuring that information the simulations provide is applicable on a larger scale requires a good understanding of the velocities and system size dependences involved. Rather than using simulation results as a “black box,” researchers must understand how the relevant mechanisms change when the scales of processes or velocities change, Szlufarska says, and this often requires using well-defined systems.
Szlufarska’s group takes a different approach. They explore chemical bonding using
ab initio (first principles) molecular simulations, which use only physical constants as input and perform calculations based on quantum mechanical principles. To explore the parameter space for the most physically probable mathematical solutions, they employ a technique known as kinetic Monte Carlo.
1 This technique has been applied extensively in various chemistry fields, including catalysis, where multiple species interact to form products. Conventional Monte Carlo methods randomly explore the parameter space to determine average static quantities, she explains. Adding the kinetic element introduces a timescale, allowing researchers to dynamically evolve the system in time based on reaction probabilities, which, in turn, are determined from molecular simulations. “If I have 100 different chemical bonding sites within the contacting interface, and we know the reaction energy barriers, then we can turn the barriers into probabilities,” Szlufarska says. “And we can say, at this time, this site bonded, and then this site bonded, I can evolve the system in time, just using probabilities. Based on the strength of these bonds, we can determine the shear strength of the interface and the force required to slide the contact.”
Evolving potentials
Interaction models, which must be developed for each chemical or physical system of interest, are another active research area. The very first models could only describe isolated atoms like argon, Martini says. Later models described the behavior of mode complex materials, starting with elemental solids like crystalline metals and evolving to multi-element systems like hydrocarbons. Early models were useful for material properties like viscosity, density or diffusion that didn’t involve chemical reactions because the harmonic spring approximations for the chemical bonds did not allow for the simulation of bond formation and breaking. Recently, she adds, some models with underlying force fields that include the formation and breaking of chemical bonds have made it possible to study some of the numerous tribological processes that happen through chemical reactions (
see Figure 4).
Figure 4. This time sequence (left to right) shows the progression of a chemical reaction between the antiwear additive tri-cresyl phosphate (TCP) and a ferrous surface (tan background). Figure courtesy of Arash Khajeh and Ashlie Martini.
Excited atomic state modeling is another area that is ripe for development, Kubo says. He notes that intense friction processes can cause the generation of excited electrons. Excited state dynamics calculations have progressed recently, he says, but only for very small molecules.
“We’ve learned a great deal from using simple potentials like Lennard-Jones potentials to look at generic phenomena,” Harrison says, referring to a well-established model, first introduced in 1931, of the attractions and repulsions between pairs of atoms. Of course, these potentials cannot accurately model a broad range of materials or chemical reactions. She cautions that fitting a set of potentials to one set of properties does not guarantee that those potentials accurately represent properties that are significantly different from those in the data sets that were used to develop the potentials.
For example, a set of potentials that is very accurate at modeling energies and structures might not work for calculating elastic constants, photon frequencies or bond breaking and making. This transferability problem can make it difficult to interpret the results of a simulation. However, Harrison notes, the most well-established potentials work well for a broad range of properties, and they can yield good qualitative results even when they are pushing the envelope on new properties. Even qualitative results are useful for identifying trends and gaining insight into process that might be occurring on the atomic scale in experiments, she says.
Szlufarska notes that in the case of more complex systems, such as silica wear in the presence of water, she uses a few different potentials, since there might not be a single one that captures all the relevant processes (
see Figure 5). She uses results of such simulations as an “educated guess” for what might be happening on the surface. She then applies a combination of physical intuition, knowledge from literature and targeted quantum mechanical simulations to determine which processes are expected to occur in reality. She adds that testing multiple types of potentials is easier now that they are implemented in publicly available software, such as the Large-scale Atomic/Molecular Massively Parallel Simulator (LAMMPS).
Figure 5. Modeling complex systems like silica wear in the presence of water often requires using several types of potentials to capture the relevant processes. Figure courtesy of Zhouhan Li and Izabela Szlufarska.
Experimental validation
Simulations are used to test theories, in conjunction with laboratory experiments.
2 “It’s really best to do simulations and experiments hand in hand,” Szlufarska says. For example, experimental measurements of friction as a function of applied load might not explain events that happen in a buried interface or processes involving microstructural evolution or chemical reactions. On the other hand, experiments can help to verify that simulations are modeling relevant processes, as well as providing accurate input for the models to work on so that they produce results that make sense. Recent advances in in situ experimental techniques have made it easier to observe processes as they are happening, which facilitates drawing parallels between simulations and experimental results.
Harrison’s group collaborated with STLE member Robert W. Carpick, John Henry Towne professor of mechanical engineering and applied mechanics at the University of Pennsylvania and his colleagues to match experimental and simulated materials and length scales for AFM tip contacts, which fall into the nanoscale and smaller range (
see Figure 6). They examined the effects of bonding on adhesion and wear
3, with simulation geometries designed to reproduce the experimental contact length, and with simulated AFM tips that were only slightly smaller than experiment.
4,5 They also examined effects of roughness on adhesion using several AFM tip sizes and materials.
Figure 6. (Left) Finite element (FE) simulation of the compressive stresses in a finite-sized diamond (111) tip at the maximum load examined. (Right) Molecular dynamics (MD) simulations of the effects of crystalline structure on stress distribution (color scale) for (top) a diamond (111) tip and (bottom) an ultrananocrystalline diamond (UNCD) tip. Colors represent stresses.7
Matching simulations with experiments remains very much a work in progress, however. “You can validate certain calculable material properties, and you can validate trends, but it’s very difficult to validate, for example, that atom X moved to the left one nanometer,” Martini says. However, model validation is necessary if modeling results are to be accepted as realistic representations of real-world processes, solidly established enough to be used as design tools. Here again, she says, progress is moving steadily in that direction.
Hardware and computing resources
One of the major obstacles to creating simulations, Harrison says, is insufficient computing power (speed and memory) for looking at systems that are large enough to mimic real-world systems and processes. Nanoscale contacts, like the length scales involved with an AFM tip on a pure-solid surface, can be modeled fairly well now with the use of parallel computing, which allows for the system to be broken up into different spatial domains that are run on different processors, she says. The simulation size and duration also are intimately linked to the potential energy function being used. Complex potentials designed to model tribochemistry are computationally expensive, while simple potentials that capture generic phenomena are not as intensive, she says.
Computer hardware can pose limitations as well. Kubo notes that it can cost 40 million yen (almost U.S. $400,000) a year for the electricity to run the MASAMUNE-IMR supercomputer. To address this issue, computer hardware and software architects have been working for more than a decade to develop “green supercomputing” capabilities.6 Supercomputers are now designed with energy efficiency in mind, and software architectures ensure that computational tasks are performed in such a way as to optimize the distance that electrons travel from one bank of cores to another.
Another problem arises when many terabytes of data must be copied from one supercomputer to another. Transferring terabytes of data created at one supercomputer center to another can take several days to a week, Kubo says, which inhibits the progress of research. Some researchers, he notes, resort to physically shipping hard disks between two supercomputer centers instead.
Artificial intelligence
Artificial intelligence (AI), including machine learning, might change the landscape of what is possible using tribological simulations, Harrison says. Humans can fit hundreds of properties when constructing potential energy functions. Computers can fit thousands of properties, which allows for a wider search of the available parameter space, enabling quantitative fits to a broad range of properties. However, she says, “The problem with machine learning is you don’t necessarily know that the algorithm is leading you to a good place. But there’s a lot of work going on to try to find algorithms that will yield good results and fit to this broader range of materials.”
In systems with many interacting features, machine learning and other forms of AI offer a possible way of sorting through the complexity to identify patterns and trends on a much larger scale than is feasible using human analysis alone. “We haven’t really figured out how to apply it yet to the microstructural evolution, but I think we will likely see significant developments in this area in the next 10 years or so,” Szlufarska says. Lubricant additives are another area that is ripe for development, she adds, because of the ability to explore a large space of chemistries. Correlation-based machine learning approaches would enable researchers to move beyond a basic understanding of mechanisms, toward designing new material compositions or systems.
Szlufarska has begun to use this type of approach in her work on metallic glasses, where a simple change in composition can affect a well-defined property, like the glass transition temperature or hardness. Because tribology necessarily involves multiple interacting processes, using AI to predict material properties and responses is more challenging. “There are so many pieces that are coupled together, but this is not the showstopper,” Szlufarska says. “I think the showstopper right now is the amount of experimental data—you have to be able to generate a lot of data to train these models.” Generating large amounts of input data often requires high-throughput synthesis of hundreds of compositions, followed by careful testing and screening. Some industry sectors, like pharmaceuticals, have developed these capabilities, but she says, “That’s a challenge in tribology because the systems are not simple.”
AI also could accelerate the development of force fields for classical molecular simulations, which can be done based on a large number of quantum mechanical calculations. Szlufarska notes that this is a relatively new and fast-developing area of research. In the past, development of a new force field was almost “black art,” and sometimes it would take years. Machine learning approaches will make such development more streamlined and more accessible to a broader community. This could enable massively parallel molecular simulations of a larger number of multicomponent systems, which have been often inaccessible to classical molecular simulations.
Multiscale models
Integrating models across different scales is another area that is still a work in progress. If you understand the fundamental mechanism of a process, you should be able to help someone solve a macroscopic problem, Harrison says. However, mapping findings on the molecular scale to the continuum mechanics that governs the behavior of say, a wind turbine gear, is nontrivial.
Molecular simulations of certain properties, like film growth rates as a function of operating conditions, might be useful for constructing models at a larger scale, Martini says. Even though it might not be possible to mesh models at different scales directly, parameters can be extracted from one model for use in another model. Tribofilm formation, a process that occurs mainly on the atomic scale, is interesting from a scientific standpoint, but it’s also very relevant to macroscale applications, she says. Here, chemical reactions between additives in the lubricant and the surfaces of mechanical components drive the formation of films that protect the surfaces. “The process can’t be captured by a bulk material property or a continuum model for heat transfer or anything like that. It literally happens at the molecular scale, so the suitable approach to studying it is necessarily on that scale,” she says.
Tribofilm formation is more difficult to model than, say, shear thinning of VI improvers, but a lot of research is being devoted to this area because “it’s something you can’t easily study with other research tools,” Martini says. “It’s something you can’t measure experimentally, because it happens between two moving sliding surfaces.” Although it’s possible to watch interactions between lubricant additives and static surfaces in the lab, this doesn’t tell you much about what happens when two surfaces are sliding past each other, with the lubricant in between.
It is not well understood how defects that begin on nanometer scales propagate to larger scales, through crystalline grains involving hundreds of thousands of atoms, eventually manifesting themselves as a fracture event on the scale of centimeters, or even meters, Harrison says. Additional complications arise for systems involving asymmetric loads, infrequent events or temperature gradients. Harrison, Carpick and Kevin Turner, chair and professor in the department of mechanical engineering and applied mechanics at the University of Pennsylvania, have collaborated on the transferability of molecular dynamics simulations to finite element modeling for AFM tips, an ideal place to start, since all of the relevant interactions are on a nanometer scale (
see Figure 6). They studied the effects of changing the characteristics of the tip using molecular dynamics and finite element analysis to study the same kinds of systems, and they developed an interpolation method to enable the extraction of a modulus from the molecular dynamics force-displacement data. The atomistic simulations demonstrated that the tip structure affects stress propagation, while the continuum simulations showed that the finite size of the tip had the least effect on contact stress.
7
Washizu’s group is investigating a technique called persistent homology
8 that takes advantage of properties like radial distribution functions that stay relatively constant at various scales. This approach creates topological descriptors that can be used in calculations of higher-order polymer structures, making it possible to distinguish and understand properties related to monomer ratios, polymer chain lengths, dielectric constants and temperature.
From simulation to application
For problems that are amenable to molecular dynamics approaches, simulations can answer questions that are difficult or impossible to answer experimentally. Washizu cites some recent research from his group that challenges the conventional wisdom that “chain matching” forms stronger films.
9
The conventional assumption was that one end of each molecule of base oil or additive attaches to a solid surface, and the chain-like molecules extend away from the surface. Thus, base oil and additive chains of the same or similar lengths should produce a smoother, stronger lubricant film that minimizes friction between two solid surfaces. Earlier simulations of these systems typically used high concentrations of additive molecules to make their behavior easier to observe, Washizu says. His group ran simulations using a realistic 4% concentration of a palmitic acid friction modifier in n-hexadecane over a timescale of nanoseconds (
see Figure 7). They found that the hexadecane base oil molecules initially lie flat on a solid iron surface, forming a dense layer.
10 The additive molecules are not able to penetrate this layer, and, thus, cannot reduce friction at the interface, until more than 10 nanoseconds into the simulation. This study also showed that base oils with branched carbon chains are better at allowing the additives to penetrate to the solid surface.
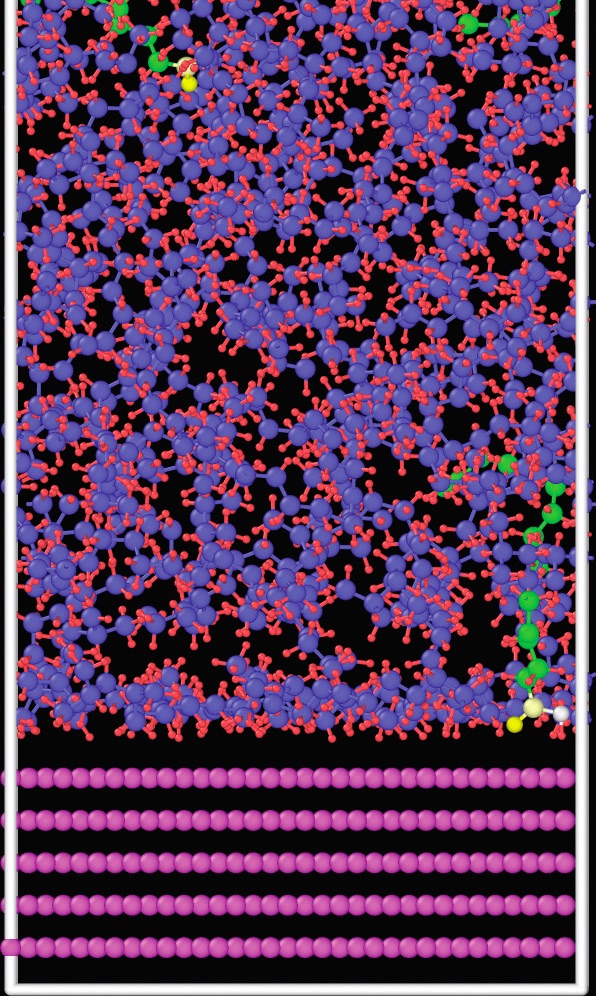
Figure 7. A layer of n-hexadecane base oil molecules (blue chains) coating an iron surface makes it harder for palmitic acid additive molecules (green chains) to attach to the surface. Figure courtesy of Masakazu Konishi and Hitoshi Washizu.
More recently, molecular modeling has been applied to electronic effects like polarization and chemical bond formation. For example, benzotriazole (BTA), used as a corrosion inhibitor on copper, protects clean copper surfaces much better than oxidized surfaces. Washizu’s research group ran ReaxFF simulations showing that fresh copper surfaces donate electrons to the BTA molecules, which chemically bond to the metal surface.
11 The excess electrons cause changes in the dipole moment of the bonded BTA surface layer, which attracts additional BTA molecules, producing a multilayered protective film (
see Figure 8). In contrast, oxidized copper surfaces form weakly bonded physisorbed BTA layers that do not attract additional layers.
Figure 8. This ReaxFF MD simulation demonstrates the polarizability changes that cause BTA to deposit more readily and form thicker films on (right) a fresh copper surface than on (left) an oxidized surface. Figure courtesy of Kohei Nishikawa.
Kubo’s research group also works to answer questions that macroscale physics approaches fail to address. For example, conventional wisdom assumes that hard materials will wear away soft materials during polishing processes. However, observation shows that cerium oxide (CeO
2) polishes silica (SiO
2), even though CeO
2 is the softer of the two materials. Molecular modeling, Kubo explains, shows that water molecules in an aqueous solution react with silica to form a softer hydrated silica layer that CeO
2 can wear away. In contrast, diamond is not effective at polishing steel, even though diamond is a much harder material. Again, models of surface processes provide an explanation. Under friction, diamond releases carbon atoms into the steel, which forms a hard carbon steel surface that wears away the surface of the diamond.
In another study, Kubo’s group studied model DLC surfaces with hydrogen atoms terminating the dangling carbon bonds. When two model DLC surfaces slide past each other under a 1 GPa load, the hydrogen atoms interact to form hydrogen gas (H
2). Even though the amount of gas generated is small relative to the overall size of the model system, it is enough to increase the separation between the surfaces and reduce the friction coefficient (
see Figure 9). At 7 GPa, the carbon atoms in the two solid surfaces come into contact and form carbon-carbon bonds, causing adhesion between the surfaces. In contrast, adsorbed species containing oxygen provide enough separation between surfaces to reduce adhesion and friction, even at GPa.
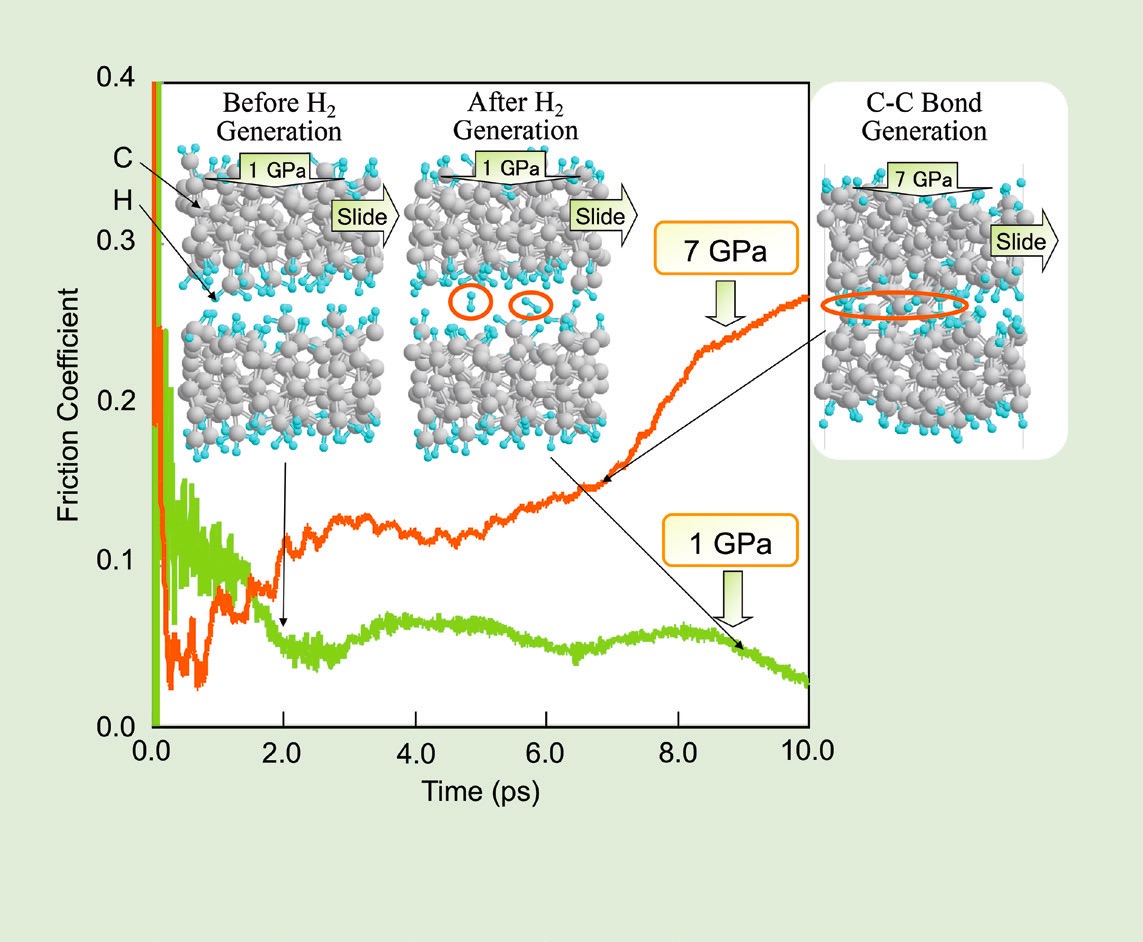
Figure 9. (Left) Hydrogen adsorbed on DLC reduces the friction between two surfaces at 1 GPa, but it cannot prevent carbon-carbon bonds from forming between the surfaces at 7 GPa. (Right) Adsorbed oxygen species increase the separation between the surfaces enough to prevent the formation of carbon-carbon bonds, even at 7 GPa. Figure courtesy of Momoji Kubo.
Kubo notes that ignoring nanoscale chemical reaction effects in macroscale systems could result in macroscale failures. A sudden increment of the friction coefficient due to chemical bond formation could be large enough to stop the engine of a moving car, he says. Researchers are only beginning to simulate tribochemical reactions of lubricant additives, he adds. For example, they have yet to generate a full simulation of the multistage process that generates MoS
2 tribofilms from MoDTC additives.
12
Recently, Harrison’s group has begun to explore the thermophysical and frictional properties of fuels in confined spaces, where interactions with the container walls play a much greater role in liquid flow properties. Previous research in this area usually dealt with single-component fluids and well-defined confining surfaces, she says. Her group is modeling various hydrocarbon mixtures to see how molecular structures affect friction. For the purposes of the simulation, they are using DLC as the solid surface of the confined space. “It’s obviously just a concept, but it’s the idea of mapping structure of the molecule and surface to the friction properties, and then being able to tailor the fuel that you use to have the desired friction properties in an engine, for example,” she says, adding that the extent to which friction contributes to a fuel’s performance in a broad range of engines isn’t well characterized. However, friction might interact with other properties such as density, thermal conductivity, viscosity, surface tension and other properties to eventually influence combustion.
Within the past decade, it has become possible to model ionic liquids (salts that are molten at room temperature), Martini says. These liquids, available in a wide variety of compositions, can be used as base oils or additives because they have good bulk fluid properties as well as good surface properties. Unlike hydrocarbons, ionic liquids preferentially interact with solid surfaces, so they provide protection under conditions that approach boundary lubrication. Ionic liquids that are miscible in hydrocarbons emerged in 2012, which opened up the possibility of using them as lubricant additives.
13 This development created an almost limitless number of cation-anion combinations to study, but it also complicates the task of finding which combinations are most promising.
At present, ionic liquids are too expensive to use as lubricants, Martini says, but understanding their fundamental mechanisms could help inform the selection of compositions that are optimized with respect to performance and cost. “That same argument applies to almost any of the other additives that we study using simulations,” she adds, noting that models can be used to understand basic processes using materials or conditions that might not be amenable to laboratory experiments because of experimental difficulties or safety concerns.
Ideally, simulations can direct research efforts along the most promising pathways, Kubo says. Understanding fundamental tribological mechanisms can generate ideas that can direct design and development. A better understanding of atomic-scale and nanoscale phenomena can result in new equations and models that incorporate the effects of chemistry and environment on friction at interfaces to give a more nuanced understanding of observable effects.
Not only can atomistic simulations capture greater complexity, Kubo says, but the knowledge they generate also can be used to construct general rules and equations that simplify representations of common phenomena.
14 These simplified representations make the results of the simulations accessible to a broader range of researchers, he adds—"You don’t need access to a supercomputer.”
REFERENCES
1.
Li, Z., Pastewka, L. and Szlufarska, I. (2018), “Chemical aging of large-scale randomly rough frictional contacts,”
Phys. Rev. E,
98, 023001.
2.
Tian, K., Li, Z., et al. (2020), “Linear aging behavior at short timescales in nanoscale contacts,”
Phys. Rev. Lett., 124, 026801.
3.
Vahdat, V., Ryan, K. E., et al. (2014), “Atomic-scale wear of amorphous hydrogenated carbon during intermittent contact: A combined study using experiment, simulation, and theory,”
ACS Nano, 8, 7027-7040. Available
here.
4.
Bernal, R. A., Chen, P., et al. (March 2018), “Influence of chemical bonding on the variability of diamond-like carbon nanoscale adhesion,”
Carbon, 128, pp. 267-276. Available
here.
5.
Milne, Z. B., Schall, J. D., et al. (2019), “Covalent bonding and atomic-level plasticity increase adhesion in silicon-diamond nanocontacts,”
ACS Applied Materials & Interfaces, 11, 40734-40748. Available
here.
6.
Loft, R. (July 28, 2020), “Earth system modeling must become more energy efficient,”
Eos. Available
here.
7.
Jiang, Y., Harrison, J. A., et al. (2017), “Correcting for tip geometry effects in molecular simulations of single-asperity contact,”
Tribology Letters, 65, Article number: 78. Available
here.
8.
Shimizu, Y., Takanori Kurokawa, et al. (2021), “Higher-order structure of polymer melt described by persistent homology,
Sci. Rep., 11, 2274.
9.
Washizu, H., Nakae, R., et al. (Nov. 7, 2020), “Mechanism of physical adsorption and chemical reactions in boundary lubrications,”
2020 International Conference on Engineering Tribology and Applied Technology (ICETAT 2020), Chiayi Taiwan.
10.
Konishi M. and Washizu, H. (2020), “Understanding the effect of the base oil on the physical adsorption process of organic additives using molecular dynamics,”
Trib. Intl., 149, 105568. Available
here.
11.
Nishikawa, K., Akiyama, H., et al. (2019), “Molecular dynamics analysis of adsorption process of anti-copper-corrosion additives to the copper surface,”
Jurnal Tribologi, 21, pp. 63-81. Available
here.
12.
Peeters, S., Restuccia, P., et al. (2020), “Tribochemical reactions of MoDTC lubricant additives with iron by quantum mechanics/molecular mechanics simulations,”
J. Phys. Chem. C, 124 (25), 13688-13694. Available
here.
13.
Zhou, Y. and Qu, J. (2017), “Ionic liquids as lubricant additives: A review,”
ACS Appl. Mater. Interfaces, 9, 4, 3209-3222. Available at
here.
14.
Yang, W., Su, Y., et al. (2020), “Reactive molecular dynamics simulations of wear and tribochemical reactions of diamond like carbon interfaces with nanoscale asperities under H2 gas: Implications for solid lubricant coatings,”
ACS Applied Nano Materials, 3 (7), 7297-7304, DOI: 10.1021/acsanm.0c01775.