Moneyball for fluid development
Dr. Nancy McGuire, Contributing Editor | TLT Feature Article August 2017
Statistical design of experiments couples a numbers-based approach with practical experience to extract the most information from the fewest experiments.
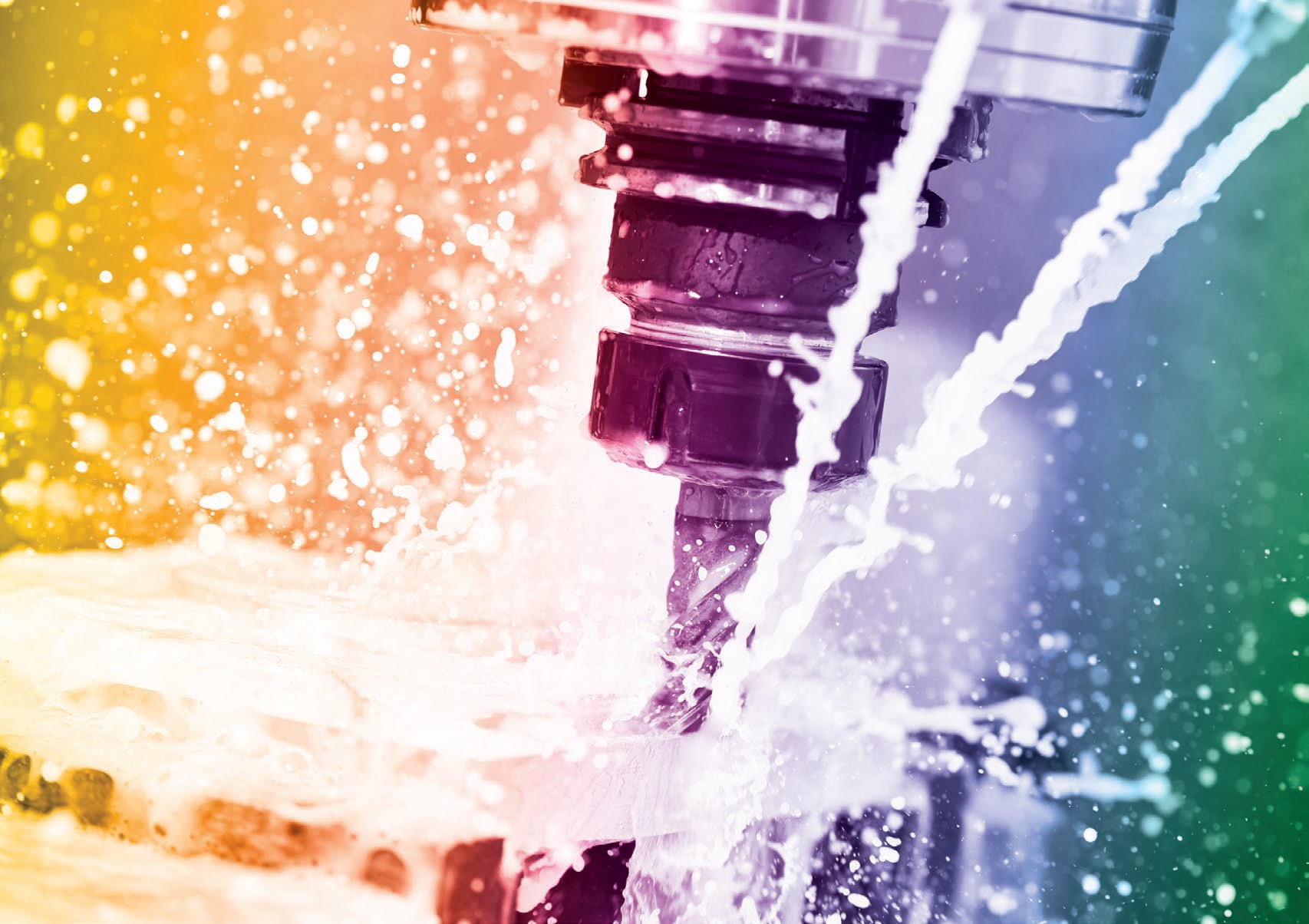
© Can Stock Photo Inc. / kadmy
KEY CONCEPTS
•
DOE identifies additives that strongly affect fluid performance, reveals interactions among multiple fluid components and optimizes fluid compositions.
•
Practical experience guides the initial setup, results interpretation and assessment of formulation changes.
•
DOE software vendors are a valuable source of training resources and applications expertise.
STATISTICAL DESIGN OF EXPERIMENTS (DOE) got its start in the 1920s when British statisticians Ronald Fisher and Frank Yates devised the method as a means of making breakthrough discoveries. Their brainchild involved lengthy and detailed mathematical calculations, and applying it required a deep knowledge of statistics. DOE use increased during the industrial boom following World War II. During the 1950s, U.S. statistician and engineer W. Edwards Deming introduced the idea of statistical quality control to industries in Japan, U.S. manufacturers having already rejected his theories.
Statistical methods for quality control and product development gained traction in the U.S. during the 1990s, with such initiatives as Total Quality Management and Six Sigma, aided by the emergence of desktop computers that took on the burden of performing the calculations and assisted in interpreting the results (
1).
The steady evolution of DOE software has made this approach more accessible to non-experts with a basic knowledge of statistical principles. Today DOE is used for such things as reducing the amount of scrap in a ski manufacturer’s operations, coming up with a new paint that won’t peel off of an aluminum tractor body and finding new formulations for metalworking fluids.
MORE INFORMATION FROM FEWER EXPERIMENTS
Statistical DOE uses numerical screening and modeling methods to extract the maximum amount of information from the minimum number of experiments. Fluid formulations developers use this systematic method to narrow their list of additive candidates, identify the formulation components that have the strongest effects on performance, examine interactions among multiple components and devise an optimum formulation window (including cost, performance and stability factors) that they can then validate in the lab.
This method is widely used in many industries (pharmaceuticals, for example), but it’s just beginning to emerge in developing lubricants and metalworking fluids. STLE-member Yixing (Philip) Zhao, senior research scientist and innovation team leader at Houghton International, Inc., in Valley Forge, Pa., notes that metalworking fluid developers often use a one-factor-at-a-time approach, sometimes called an Edisonian approach. This method is good for initial screening efforts but often falls short during optimization, he says. It’s not uncommon for a metalworking fluid to have 15-20 components, and many of them interact with each other (
see Figure 1).
Figure 1. The relationship between additive packages and fluid properties and performance is complicated. For example, additives that improve lubricity (solid line) also might affect emulsion stability and foaming (dashed lines). (Figure courtesy of Philip Zhao, Houghton International, Inc.)
“This industry really needs DOE,” Zhao says. “We’re a small industry with limited resources and a reliance on old knowledge. There’s a reluctance to use DOE, but this could revitalize the area and bring more efficiency.” He notes a certain reluctance to part with the old methods, but DOE lets developers serve customers better and discover new chemistries as well as confirming whether new additives will work.
“DOE can help you identify correlations among multiple additives and between additives and properties,” he says. “Testing one variable at a time requires a lot of experiments and doesn’t show you synergies and correlations.” In addition, he notes that DOE reveals new correlations, cuts down on the number of samples you need to test and keeps you from running in circles when interactions among additives introduce complexity (
see Figure 2).
Figure 2. DOE software provides several capabilities that bridge the gap between individual components and the desired properties of the resulting fluid formulation. Laboratory experiments serve as input. The software identifies correlations, which are statistically analyzed and used to find optimal compositions. (Figure courtesy of Hoon Kim, Chemetall.)
Using DOE doesn’t diminish the value of personal experience, Zhao emphasizes. You still need to do the initial testing. “We don’t run hundreds of samples like the pharma industry does,” he says. “You have to pick your samples for the initial work and understand what the results actually mean and how significant the effects are.”
STEP BY STEP
Dan Trauth, process research leader at ANGUS Chemical Co. in Buffalo Grove, Ill., was already an experienced DOE user when he first became involved in fluid formulations. He had used the method in optimizing plant operations for chemical manufacturing. In the plant, he used DOE to understand product quality, capacity and impurity levels: “I can dial things up or down,” he says. Fluid formulations are different, though. “If you’re developing a mixture, you can’t just keep adding stuff. The components are not entirely independent, and the formula composition must add up to 100%.”
In developing formulations, historically, you would use trial and error; you would test everything, Trauth says. But it was hard to tell whether the differences you saw were real or due to measurement errors. “Once you start adding components, the number of variables gets very large very fast,” he says, noting that testing eight variables gives you 2
8 or 256 experiments, if you are doing a complete DOE including high and low values. To minimize the number of experiments, he says, “You want to test the variables with the most impact.” He adds that screening experiments narrows the number of variables so that you can test the broadest range possible for each variable without causing the experiments to fail.
Thus, the typical DOE process for fluid formulations involves two or three phases. In the first phase, developers run screening tests in the lab, replacing additives one at a time and drawing on their previous experience. They enter the results of their lab tests into the DOE program and run a screening analysis to see which additives have the strongest beneficial effects on overall fluid properties. Additives that affect the results very little—or that have a negative effect—can be eliminated from further consideration at this point. The initial screening run also gives an indication of which parameters are the most effective ones to optimize. The results of the initial screening run narrow the problem down to a manageable set of variables.
Once the analysis of the screening run is complete, Trauth says the developer defines an objective function to use in the optimization run. This is where the developer’s main goal comes into play. Are you going for low cost? Best performance? The DOE software will calculate the formulation range that maximizes what you’re going for. Formulations in this range are then tested in the lab to validate the results.
Zhao chooses the three or four most effective additives for the next phase of the statistical analysis. He uses a factorial DOE method (testing all combinations) to identify correlations among the additives and optimize the formulation. He notes that in some industries, like pharmaceuticals, where the number of variables tends to be much larger and the research can be at a much earlier stage, a three-step process is used: screening, identifying correlations and formula optimization. For his purposes, however, he finds it more efficient to combine the correlation analysis and formula optimization into one step.
Hoon Kim, senior principal R&D scientist at Chemetall, a part of BASF Group based in New Providence, N.J., develops new metalworking fluid products with an emphasis on fluids for use with aluminum. He spends about half his time developing formulations and the other half developing new additive technology. Part of the new additive technology work addresses environmental issues like finding replacements for chlorinated paraffins.
Kim uses a similar two-step DOE process. “Screening lets us look at multiple factors (more than six, say) and see which ones have the strongest effects. If we’re only studying two or three factors, we sometimes skip the screening and go right to the predictive analysis,” he says. Predictive DOE is where he takes the most influential factors, finds the correlations between them and builds his model. This is where he does the optimization work. Kim also emphasizes the importance of setting parameter limits and specifying a goal for the optimization in terms of cost, performance or some other criterion. “The computer finds an optimal formulation based on those criteria,” he says.
Specialty chemical companies might skip the screening altogether, Kim says, since they produce individual chemicals and are mainly interested in optimizing the production process. Fluid formulators, on the other hand, are dealing with multi-component systems, and they need to narrow the factors they study to keep the number of experiments manageable.
Kim and his colleagues have used DOE for a variety of projects. For example, they developed a new metalworking fluid formulation using 40 lab screening experiments as input to the DOE program to come up with the one final formulation. “We would have had to test hundreds of formulations otherwise,” he says.
In another study, they compared the anti-staining properties of a fluid additive on aluminum alloys (
see Figure 3). After the initial screening tests, DOE helped them optimize the concentration of the additive to provide the best stain protection without compromising the overall fluid performance.
Figure 3. DOE analysis is used to optimize the concentration of fluid additives. Here the effects of varying the concentration of an anti-staining additive are validated using aluminum alloy test strips. (Figure courtesy of Hoon Kim, Chemetall.)
Kim also cited the example of a new extreme pressure (EP) additive technology that his group developed (
see Figure 4). They used DOE to see which chemistries were most effective at improving lubricity. “We put in all the data and the computer came up with the best formulation,” Kim says. They validated the optimal formula that the DOE recommended in the lab, “and it works!” he says. “The computer generated the formulation, not us!”
Figure 4. Multivariate plots show the linear correlations between multiple variables simultaneously. Elongated elliptical shapes indicate a strong correlation between variables, while a broad round ellipse means a weak or no correlation. Here mixture design DOE was used to evaluate the lubricity performance of two new lubricity agents (EP and LE) compared to the current technology (PP). Only EP shows positive correlation with lubricity on steel (yellow squares). Parameter estimates from the DOE indicated a potential synergistic interaction between EP and LE on aluminum (blue squares). This result indicates that the new EP and LE technology can improve the lubricity performance of the current formulation. (Figure courtesy of Hoon Kim, Chemetall.)
EXPERIENCE STILL COUNTS
DOE doesn’t do the science for you, however. Software packages like Aspen incorporate known physical phenomena and physical property databases into their analyses, but DOE analysis is firmly based in laboratory testing. “You have to set up the screening, and that requires knowledge,” says Trauth. The DOE provides the math to help you arrive at conclusions. Manufacturers can’t make huge changes in the plant or try out every possibility, so DOE lets you extrapolate from your initial screening tests, he adds.
Experience is helpful in picking a set of variables for initial screening, but it really comes into play when it’s time to interpret the results and begin to optimize a process or formulation. “Look at the error bars; how much uncertainty is in the results?” asks Trauth. This is a good indicator of whether results are significant or merely a result of experimental uncertainty. If the experimental error is too large to derive a meaningful result, “that’s when you do a second set of DOE calculations over a tighter range.” If it’s really cheap and fast to do the experiment on the fly, then another set of lab tests can provide clarity, but “if it takes a significant amount of time or effort to do the experiment, then DOE is more efficient,” he adds.
Experience also is valuable for getting a feel for what should work and knowledge of what just doesn’t work, Trauth says. At a former job, his employer tried using high-throughput research. This approach, in which you use automated lab analysis instruments to test every possible permutation, is the opposite of DOE, which seeks to narrow down the number of variables. They used automated analysis to run hundreds of tests, but it turned out that DOE was better at producing the needed information.
CASE STUDY: A TALE OF THREE ADDITIVES
Zhao and his colleagues used DOE to examine the effects of various additives on soluble oil cutting fluid emulsions. Their objectives included improving emulsion stability in very hard water, reducing the tendency to foam and maintaining good blooming (emulsion spreading) characteristics.
They compared three nonionic surfactants, each having a different hydrophile-lipophile balance (HLB, a measure of a surfactant’s ability to form emulsions) to see if high-HLB surfactants caused more foam. For the screening phase of the DOE, they compared nine formulations by injecting cutting fluid concentrate into hard water and recording the emulsion travel distance with time, using a Turbiscan™ instrument (
see Figure 5). They also compared the stability of the emulsions over a 25-hour period (
see Figure 6) and the foaming properties of each emulsion (
see Figure 7). They entered the results into the DOE program to find correlations between formulations and properties and to generate an optimal formula.
Figure 5. Results of an emulsion blooming test using nine combinations of three additives (top table shows mixture compositions). Formula 2 formed an emulsion that quickly reached the bottom of the cylinder. Formulas 3 and 6 only formed emulsions in the top 2/3 of the cylinders. The travel distances for each sample (bottom rows of the table) were used in the DOE analysis to find correlations between additive combinations and blooming distances. (Figure courtesy of Philip Zhao, Houghton International, Inc.)
Figure 6. Turbiscan™ results for the group of samples shown in Figure 5. High backscattering intensities (BS%) indicate less breaking up of the emulsion. After 25 hours, significant changes are apparent in the emulsions at 50 C. Formulas 5 and 6 showed the least amount of change, indicating that the high-HLB emulsifier improves the stability of the emulsion. (Figure courtesy of Philip Zhao, Houghton International, Inc.)
Figure 7. Analysis of variance (ANOVA) on the results of foam tests shows that all of the p-values are less than 0.05, indicating that all three emulsifiers and all of their combinations significantly affect the foaming properties of the hard-water emulsions, either increasing or decreasing the tendency to foam and the stability of the foam. (Figure courtesy of Philip Zhao, Houghton International, Inc.)
Analysis of variance (ANOVA) testing from the DOE program showed that the medium-HLB emulsifier significantly improved blooming, the low-HLB emulsifier made it significantly worse and the high-HLB emulsifier had no statistically significant effect. The analysis also showed that mixing either the medium-HLB or low-HLB emulsifier with the high-HLB emulsifier produced synergistic effects: the medium-HLB mixture got better (however, it turned out not to be a significant improvement) and the low-HLB mixture worsened.
After doing a DOE optimization run to balance the effects of emulsifier HLB on blooming, emulsion stability and foam (
see Figure 8), the Houghton team devised an optimal formulation that was significantly better than their control formula (
see Figure 9). This formulation was then validated in the lab (
see Figure 10).
Figure 8. DOE helps developers select the optimization parameters that have the greatest effect on a fluid’s performance. They enter concentration ranges for each additive into the factor section and the desired ranges of specific properties into the response section. The upper and lower weights and the importance values shown here are default values for the DOE program, but these could be adjusted as well. (Figure courtesy of Philip Zhao, Houghton International, Inc.)
Figure 9. The DOE software arrived at a formulation that produced the best combination of blooming distance, foam dissipation and emulsion stability. (Figure courtesy of Philip Zhao, Houghton International, Inc.)
Figure 10. Lab tests validate that the optimal formula produced by the DOE analysis performs as well as or better than the control formula for blooming properties (left), resistance to foaming (top right), and emulsion stability (bottom right). (Figure courtesy of Philip Zhao, Houghton International, Inc.)
GETTING STARTED
Unlike the pioneers of DOE, today’s fluid developers don’t need to be experts in statistics to use the methods effectively. However, they still need a basic knowledge of statistical principles and the ability to see what the results mean.
Learning to use DOE is like learning to drive a car, says Kim; in the beginning, you’re nervous. But once you get comfortable using the car, it can take you a long distance very quickly. You don’t need to know everything about the car or how to build one to be a good driver. But it still requires some skill from the driver.
Anyone wanting to get started with DOE should check the software vendor Websites, Kim recommends. The vendors offer free tutorials on their Websites that are very detailed. You can go to training sessions geared to your level of experience, he says.
Zhao recalls, “I only had one stats course in college, and I still remember a few basics, but you don’t need much.” Over the course of his career he has used three software packages. Some software is more powerful but difficult to use, and some software assumes that you are familiar with more technical terms. “Don’t be afraid to use the software, but do get the training,” he recommends.
Zhao says that with training, you learn to interpret the results. On a basic level, you can examine p-values and make sure that they are less than 0.05. This typical cutoff point tells you there’s at most a 5% probability that the differences you’re seeing are caused by experimental error or uncertainty. (Put another way, there’s at least a 95% chance that the differences you’re seeing are real.) “Even if a correlation is what you want, if it’s not statistically significant, don’t use it or re-examine it,” Zhao says.
“If you don’t know what you’re doing, statistics can lead you astray,” says Trauth. “I took a stats class in college and used statistics in grad school and in my career. Six Sigma really got me into it,” he says, referring to his time at Dow Chemical Co. “When I started, we didn’t do much DOE, but Dow went in really big for Six Sigma” (a set of management practices aimed at reducing inefficiency and errors to an absolute minimum). This effort included a lot of statistical analysis, and employees received training in the processes and methods, he says.
Trauth is one of two resident DOE experts at ANGUS, but even so, they rely on help from the company’s software tech support people. Most of his experience is in using DOE for manufacturing processes, but now he works with fluid formulators as well. He recalls that there was some resistance at first to using DOE for formulations. “The thinking was, if you stay out of the stats box you can be more creative,” he says. Part of this was a tendency on the part of company chemists to look for big changes, but his experience as an engineer taught him that small but significant improvements can add up to substantial cost savings.
Kim, Trauth and Zhao all noted that the software vendor’s tech support people were an important source of information and advice. Often, these tech support staffers are experts in a particular field, and they have a deep knowledge of the software and its applications. In addition, they gain experience from working with several client companies on their applications. Although they can’t share specific information between companies, they are likely to know how to deal with certain situations, drawing on this experience.
Software vendors also sponsor user groups and forums, online and at conferences, where users can share their know-how with one another. This is especially helpful in smaller operations where there might not be an opportunity to do this kind of sharing in-house.
For example, Kim is the only person in his group to use the JMP software package. “I make use of JMP’s online user community,” he says. “They have blogs and online information exchanges where more than 8,000 members interact. They also sponsor live conferences called Discovery Summits that are forums for users to share their experience and give presentations on how to use the software for various applications.”
Zhao and Trauth aren’t as involved in software user communities, but they do make use of their own companies’ in-house tech support staff as well software companies’ tech support staff. They recommend the workshops that the software companies sponsor as the best place to begin DOE training. Some of this training is online in the form of tutorials and webinars, and some is offered in person. Larger companies might have in-house user groups and training as well.
AN EMERGING CAPABILITY
Software and statistics are all very well and good, but can statistical DOE help you improve formulations in the real world?
Trauth says that ANGUS Chemical makes four compounds, and sales for one of them grew faster than for any of the others. “You want to make more of what sells,” he says. The reaction that made the compound was very exothermic, and it was hard to control the temperature and pressure. DOE let Trauth and his team optimize the reaction conditions. They came up with a model that showed they could control the temperature more easily if they used dilute rather than concentrated nitric acid in the reaction. His colleagues were skeptical, but “it worked beautifully!” He adds, “DOE can open up spaces you wouldn’t imagine would work.”
Kim notes that DOE is an emerging technique in the metalworking fluids community. Fluid formulators are starting to use it more often, but it’s still not commonly used. This is a mature field, he says, and senior people would rather trust their own experience. “But once they see what DOE can do, they see that it works,” he says. “I want to see more people use DOE!”
REFERENCE
1.
A Quick History of the Design of Experiments (DOE), STAT 503: Design of Experiments, Penn State Eberly College of Science. Available
here.
Nancy McGuire is a free-lance writer based in Silver Spring, Md. You can contact her at nmcguire@wordchemist.com.