Predictive maintenance
TLT Sounding Board March 2020
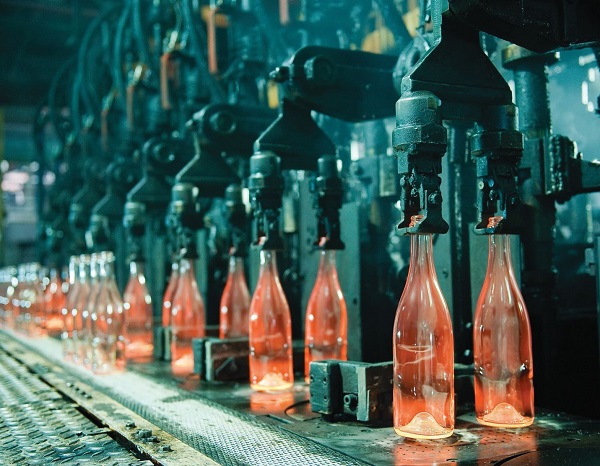
Executive Summary
The best news from this month’s survey came from readers suggesting that, after decades as lower-priority items, condition monitoring and predictive maintenance are getting more attention from senior management. Survey respondents say managers in greater numbers are understanding the high price of equipment failures and the cost of process interruptions. “It was less important 30 years ago, now it’s viewed as an absolute necessity,” said one survey respondent. Readers add that artificial intelligence (AI), online real-time sensors and the Internet of Things (IoT) are expanding their roles in condition monitoring, as they generate results faster and reduce the need for full-scale labs. But there is still room to grow for most end-users as these technologies continue to be incorporated into the condition monitoring process. Said one respondent: “There’s potential, especially with AI, but the promise is way off of where reality is right now.”
Q.1. Describe the most significant change in condition monitoring and predictive maintenance you’ve seen during the past year.
Advances in identifying the metallurgy of individual particles in oil, not just overall concentrations.
The use of high-speed cameras to show vibration.
Artificial intelligence.
Acoustic monitoring of engines.
In-line/real-time monitoring sophistication has improved.
Commercially affordable design and implementation of real-time scanning electron microscopy in particle size determination and analysis.
The testing of incoming product cleanliness was conveyed by a customer; this is where the condition monitoring truly starts!
Online submission of data which, in turn, speeds the results turnaround by >50%. We hear much about enhanced testing and equipment, but human involvement is still required.
AI used to predict failures.
It has become a requirement for many of our customers to attend training.
Thermal tools to predict wear are more in use.
Remote health monitoring and real-time oil analysis sensors.
Condition monitoring using online real-time monitoring (IoT) using the CMMS as an interface to raise request for values going out of setpoints.
The increasing use of portable and in-line measuring devices in equipment and the increase in knowledge in the culture of lubrication and reliability maintenance professionals.
Software-based AI involvement.
New and sophisticated instruments for precise testing.
Sensors are getting smaller and cheaper.
As more sensors become wireless and “stick-on” and inter-connected, we will see a great improvement in equipment reliability.
Which is the most important goal of predictive maintenance?
Improve equipment availability 54%
Reduce maintenance cost 46%
Based on responses sent to 15,000 TLT readers.
It is not limited to the past year that the availability and affordability of sensors and handheld devices seems to be accelerating. Yet, using those consistently for predictive maintenance is lagging.
Companies that we deal with have taken a more proactive view during the past year. For many of them, this is a new initiative. They have learned the hard way that just reacting can actually cost more money. One company had a machine that was critical to its success. Management waited years to address it and got into a situation where the machine was close to failure. They contracted to have the fluid changed and the PM work done to the tune of $250,000. Had they reacted sooner, the cost would have been way less.
The automation and categorization (barcoding systems) that have taken the place of time-consuming data entry.
Ultrasound is becoming more common.
More companies are starting to switch to these methods over the traditional time or routine-based maintenance.
Online constant monitoring of lubricant quality through cell towers.
Vibration monitoring on all types of rotating equipment which is now more cost effective due to reduced cost design.
The use of ultrasonic sensors in parallel to lubricate the friction nodes of devices.
The use of AI diagnostics for the incorporation of all machine health data (oil analysis, vibration, telematics, sensors) into a single integrated platform.
Used oil analysis to analyze the break-in course for equipment. Monitoring of lowspeed equipment using oil analysis.
Need to align with OEMs for diesel/natural gas engines for minimum/maximum wear metals for alerts.
The connection between simple condition monitoring by local sensors/devices goes further to a connected network of sensors in a machine and a cloud system in the background (IoT shapes more and more).
Merging of service companies.
The most significant change in condition monitoring and predictive maintenance I have witnessed or heard about during the past year is that more companies are realizing the true value of these methodologies in increasing equipment uptime and availability.
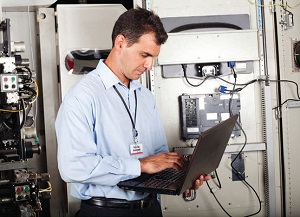
Q.2. Please describe roadblocks preventing the use of predictive maintenance.
Budget restrictions not allowing for either the personnel to shepherd a program or for the tools/training to properly run a program.
Management’s lack of understanding of the cost of failures and the cost of process interruptions.
Time and lack of interest.
Too many sources of conflicting guidance on limiting values.
Budget constraints (short sightedness!).
The (perceived) high initial cost.
The thought in industry that still supports the lack of validity in condition monitoring is the biggest roadblock.
Personnel and allocating the time to process samples timely and accurately.
Funding and outage durations to allow device installations. The culture attitude of, “We haven’t had it for 100 years and haven’t needed it.”
Older maintenance supervisors not wanting to accept change and use new procedures.
No space for mounting control sensors, unconscious of inexperienced designers to predict space for such sensors, low susceptibility of new devices to predictive maintenance.
The cost of CMS on wind turbines (my industry) is completely out of line with the actual savings offered. I’ve never seen a product that I projected to have a positive ROI for my company.
Depending on the process, they start from the investment planning of portable or online devices when new equipment is to be purchased, then buy them as limited as possible to have less investment cost.
The high cost of updating the equipment and adapting cutting-edge technology.
Sometimes they prefer to work with the essentials.
In general, the high cost of access to available technology.
Software interfaces.
People turnover.
Who is best qualified to conduct preventive maintenance and interpret the results?
Independent third-party service companies 16%
Internal staff 61%
Equipment OEM 24%
Embedded component/equipment software 29%
Component vendors 16%
Based on responses sent to 15,000 TLT readers. Total exceeds 100% because respondents were allowed to choose more than one answer.
Reluctance to spend the money and time to retro fit. It is criminal for instance, that there are so few wind turbines that are fully instrumented with appropriate sensors to gather condition information. A wind turbine tech should be able to monitor the performance of bearings and gears, etc., from the ground before climbing the tower.
Maybe the largest roadblock is baseline data that accounts for the location, environment, user, and duty-cycle so as to minimize false alerts and the frustration and inefficiencies that go along with false alerts.
Lost local knowledge in end-users themselves.
New equipment takes time to build a trend, and predictions cannot be made until that time. Another roadblock with older equipment is that, in some cases, a baseline is not present to compare with the used. This results in inaccurate data and untrustworthy predictions.
Costs, availability of the technology, awareness, training and willingness of the staff and data security are some of the challenges in implementing and use of a reliable predictive maintenance.
Myopic equipment managers not wanting to spend money unless equipment is broken.
Overcoming the lack of lubrication knowledge or stigmas around lubrication. For example, I must change the oil in my car every 3,000 miles because the car light/sensor tells me to.
Management resistance to spending capital and breaking old habits.
OEM alignment. Sometimes difficult to align in terms of technologies—differences in goals and targets.
Being able to schedule enough equipment down time to perform the needed maintenance.
Lack of understanding of the fundamentals of predictive maintenance.
Lack of management of predictive maintenance programs.
Cost.
Customer resistance to change maintenance practices. Lack of understanding of failure to properly maintain systems on the part of customers.
You have to have a mindset whereby you have to be diligent with it and maintain good records.
In the classes I hold at various companies, I always see the weakness of lubricant knowledge among organizational managers and personnel. Not paying attention to the depth of the problems.
False actions/alerts. Predictive maintenance is still not precise enough to predict remaining life time of a system/component/tool or bearing. In new machines, the accuracy seems to be better. In older machines, the censoring is often not sufficient to make proper prediction for the system.
Machine guards.
For old equipment, one of the roadblocks is communication with old data systems.
Q.3. Please describe the impact of the Internet of Things and artificial intelligence on condition monitoring and predictive maintenance.
Still low. It’s kind of like selling a Rolls Royce to someone who doesn’t know how to drive and has a budget of $1,000.
It makes information transfer better, but its misuse tends to bury people with data that may or may not be of value.
Lessening the need for full-scale labs.
Big increase in interconnectedness readily allows both Big Data and microdata analysis!
Broad implementation of real-time monitoring and failure prediction due to reduction in prices.
Extremely powerful in noticing and highlighting the influence of the minute details and aid in proper diagnosis.
The internet has boosted the knowledge of people needing to find possible similar issues with equipment.
Increasing the speed and amounts of transmitted signals—the drawback of frequent training for operators of equipment due to the rapidly changing software and hardware configurations.
There’s potential, especially with AI, but the promise is way off of where reality is right now.
Measures details and we are able to stream real-time data as the equipment is running in a remote location.
They help document and refine the technical information data for analysis.
Huge impact. Data processing is in a new era. Advance pattern recognition and data modelling have changed the philosophy of conventional predictive maintenance.
We are just scratching the surface with the use of IoT and AI for condition monitoring and predictive maintenance. However, as sensors (vibration, temperature, etc.) become smaller, cheaper and wireless, we will see the benefit of AI to handle more and more condition monitoring information and format it into real actionable intelligence.
In the future, this information could even be used by the OEM to improve the reliability of even individual components in machines.
IoT and AI have great potential to improve condition monitoring and predictive maintenance and its benefit for reducing unscheduled interruptions and minimizing downtime. But in many (most?) situations, insufficient knowledge exists regarding all the potential failure modes and range of operating conditions, leading to false alerts.
With the companies we deal with, this is still something that is out of reach.
IoT and AI have expedited the processes involved in predictive maintenance.
Results are obtainable much faster.
IoT and AI are crucial for the condition monitoring and predictive maintenance connecting the equipment on the cloud.
The potential expenses as well as data and equipment security challenges should, therefore, be of major consideration.
The internet has good information, but many forums are the blind leading the blind with bad misinformation.
It is helping to bring awareness to people who truly want to know/understand more.
Potential for faster response and increased data analysis to drive more efficient operations, particularly on a large fleet.
It is huge and is what has brought down the cost to implement.
Earlier detection of incipient failures has significant impact on lead times to failure and allows for proper planning and scheduling. The P on the P-F curve is detected earlier than any physical CM methods such as vibration monitoring and oil analysis via sampling. This results in significant savings in the mining industry.
There is also a significant reduction in safety risk as the human-machine interface is reduced.
AI for machine learning models has allowed for more data to be considered when diagnosing machine faults.
More information can be found on the internet these days so condition monitoring information is more readily available.
Unfortunately, at present our company does not pay much attention to IoT.
AI can help increase a remaining lifetime in a specific machine due to machine learning capability. This can close the gap between traditional life time calculations and real failure modes in a specific machine, which has its own boundary conditions. Usually you don’t know all parameters in a system you need to make a realistic life time prediction. AI/machine learning does not need this detail. It just learns out of the given system step by step.
I believe it will have a monumental impact on condition monitoring and predictive maintenance. This is why some have coined the term the 4th Industrial Revolution. In a world where everything seems to keep getting faster, these will definitely be a game changer. In the past, it may have taken weeks or even months before an underlying fault condition manifested itself. However, now we are looking at minutes and hours.
Editor’s Note: Sounding Board is based on an informal poll of 15,000 TLT readers. Views expressed are those of the respondents and do not reflect the opinions of the Society of Tribologists and Lubrication Engineers. STLE does not vouch for the technical accuracy of opinions expressed in Sounding Board, nor does inclusion of a comment represent an endorsement of the technology by STLE.