Complexity in oil analysis information: Part IV
Jack Poley | TLT On Condition Monitoring May 2012
Enter the intelligent agent.
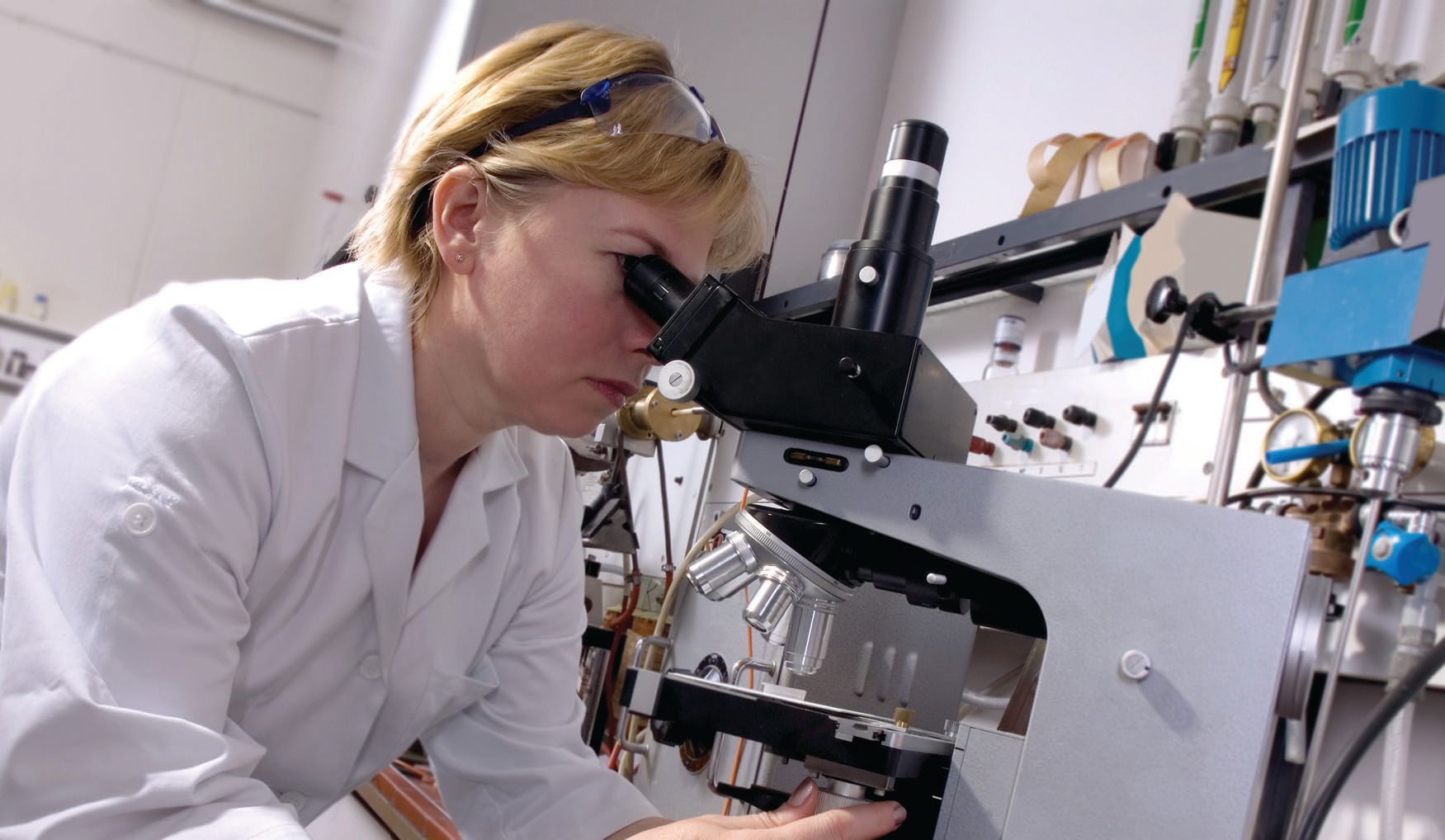
www.canstockphoto.com
I HAVE COVERED THE MAIN ASPECTS OF, OR INFLUENCES ON, DATA RATING in the last three columns, culminating with the table shown here. The range of knowledge we amass on the component is spanned as follows.
Minimum: We must identify the component at least in terms of its type (hydraulic, diesel, gearset, etc.) before we dare build limit and trending tables at even basic generic levels, and we’ll have to provide for wide tolerances in this very generalized arena because we will not know the subtype (hydraulic, axialpiston pump; hydraulic, gear; hydraulic, vane). And everyone knows to jump all over coolant additive chemistry (Na, B, K, e.g.) for diesel engines. When these elements accumulate in the oil together—wait diesel engines have sub-types, too! How many coolant leaks will you detect in an air-cooled diesel? How many air-cooled diesel MFRs can you recite?
Maximum: We have all the information available as defined in the table. Given possession of all the aspects in the table, we have the makings of very specific limits and trends algorithms that will provide trustworthy flagging and data rating (always assuming we have at least 30-50 precise examples and that those examples, if possible, come from at least a half dozen or so separate components of the same ilk).
In a typical condition monitoring setting, we rarely have
all such data and, sadly, we are often operating on minimum data. And
really sadly, some of that data are at times just plain incorrect, owing to typos or other carelessness such as multiple ways of expressing the same value for a field (CAT, Caterpillar, Caterpiller).
This goes back to the point I made a few columns earlier where, not anticipating a need to have a solidly seeded and maintained database, equipment owners and maintainers were satisfied to have a “No. 2 Compressor, South Wall” as a component description, with all involved knowing its manufacturer and often much more than that. No one was thinking about CM or oil analysis at that time. Suddenly a decision is made to adopt this valuable practice, and highly useful, often necessary information is then lacking when the .csv file comes in from the site’s database. I guaranty you that most of this is rarely corrected to a high quality level once this information is embedded. Ultimately it’s everyone’s fault.
Summarizing this somewhat anecdotal set of examples and the crux of the previous three columns, data rating becomes increasingly more accurate and reflective of a given component when the best effort is made to gather and post full and accurate information. It is further valuable to assign a hierarchy to this information as suggested below in order to establish rules for evaluation:
1.
Equipment Type. Where the component resides, e.g., a ship, a plant, a bulldozer, a truck, a drilling rig, etc.
•
Equipment MFR.
•
Equipment Model.
2.
Component Type. This is required and indicates where the lubricant sample came from.
•
Component subtype. This often is required for meaningful evaluation (note the hydraulic example cited earlier).
•
Component MFR. This often is not the equipment manufacturer.
•
Component Model.
3.
Application. Oil and gas, aviation, marine, off-highway, industrial, etc.
•
Sub-Application (offshore, oil and gas, off-highway, forestry, etc.)
4.
Wild Card. If more differentiation makes sense, it is accomplished here.
5.
Lube MFR and brand. Knowing the oil company is useless unless its product is also identified.
•
VIS Grade.
6.
Filtration manufacturer and brand.
•
Filter Type (full-flow, bypass, screen, centrifugal, etc.)
•
Filtration micron (µ) or beta rating (ISO Particle Count Code target in place).
Given that we amass as much trustworthy information as possible, and given we’ve used reasonable mathematics to create limits and trend tables, and once the mechanisms are in place to continue to update such data on a periodic basis, it should be relatively easy to maintain limit tables. Arriving at reasonable limits for test results is not highly difficult but does require consistent, careful gathering and collating of information on an ongoing basis. Serious CM practitioners need to make this a habit. It’s not optional if strong financial benefits are expected. Preparation, as so often is the case, is the key to success.
Much harder, however, is the task of furnishing a reasonable opinion and recommendation as to what to do, thus we finally come to the most difficult aspect of OA: The Evaluation, the Comment or Advisory that we will issue.
If you are an evaluator in a commercial laboratory viewing samples from all sorts of components from all sorts of machinery types, you will be hard pressed to memorize all the possible permutations that can arise; in fact you cannot expect to do it. Even if you’re confining your scope to specific types of applications (or if you’re operating in a private lab that services specific types of machinery), the pressure to retain combinations and references is enormous. And if you have ever evaluated a “South Wall Compressor” in your career, let’s hope you guessed correctly – you likely had no idea what you were looking at unless you were employed at the plant where that component resided.
What if you are a report recipient? You may have the expertise (because you know your equipment) to perform a competent evaluation, but wouldn’t it be simpler if an accurate suggestion were available initially such that all you had to do was tweak it, if at all? Most report recipients have many more responsibilities than reading oil analysis or other CM data.
No doubt you’re familiar with your machinery and its function, as well as most of its idiosyncrasies. What about the lubricant? Do you have a full understanding of that lubricant’s properties and characteristics in-service? Do you know what to say and do when additive metals, wear metals, particle counts, acid numbers and viscosities move around? Can you spot the pertinent relationships and separate them from those not so indicative? If so, please pass this information down to others before you retire. Expertise like yours is increasingly rare.
If, however, you aren’t fully comfortable with both the machine and the lubricant interaction, or even if you are comfortable but want to provide a margin of safety while developing a consistent approach to evaluation, then you might want to employ an intelligent agent using not only limits and trending but pattern recognition, as well. Software such as this is increasingly more accessible and can make a marked difference in what you extract and the ROI gain from your CM program.
Pattern recognition is not a new concept by any means, but it is not always a fully exploited technique in data monitoring where CM is concerned. And although pattern recognition is a sound analytical device, it is not effective unless it’s coupled with domain expertise. In truth the very best evaluations are collaborative in nature.
Statistical experts, usually using computer-generated algorithms, identify patterns. Domain experts then inject knowledge into the identified pattern, which leads to a rule that fires a message containing an opinion and possible recommended action when such patterns occur. Repeated refinement, nuancing and exception allowances, vetted by feedback from the maintenance site, confirms or, at least, bolsters trustworthiness to the opinion.
A particularly important task of the domain expert is to assign importance or weight to particular patterns and opinions that are more likely indicative of actual conditions, separating them from patterns that are less likely to be indicative.
In our next article, we’ll explore a variety of patterns in various venues and applications.
Jack Poley is managing partner of Condition Monitoring International (CMI), Miami, consultants in fluid analysis. You can reach him at jpoley@conditionmonitoringintl.com.
For more information about CMI, visit www.conditionmonitoringintl.com.