Strategic oil analysis: Selecting alarms, setting limits
Mike Johnson & Matt Spurlock | TLT Best Practices November 2009
Proper planning can prevent severe damage in the event of a failure.
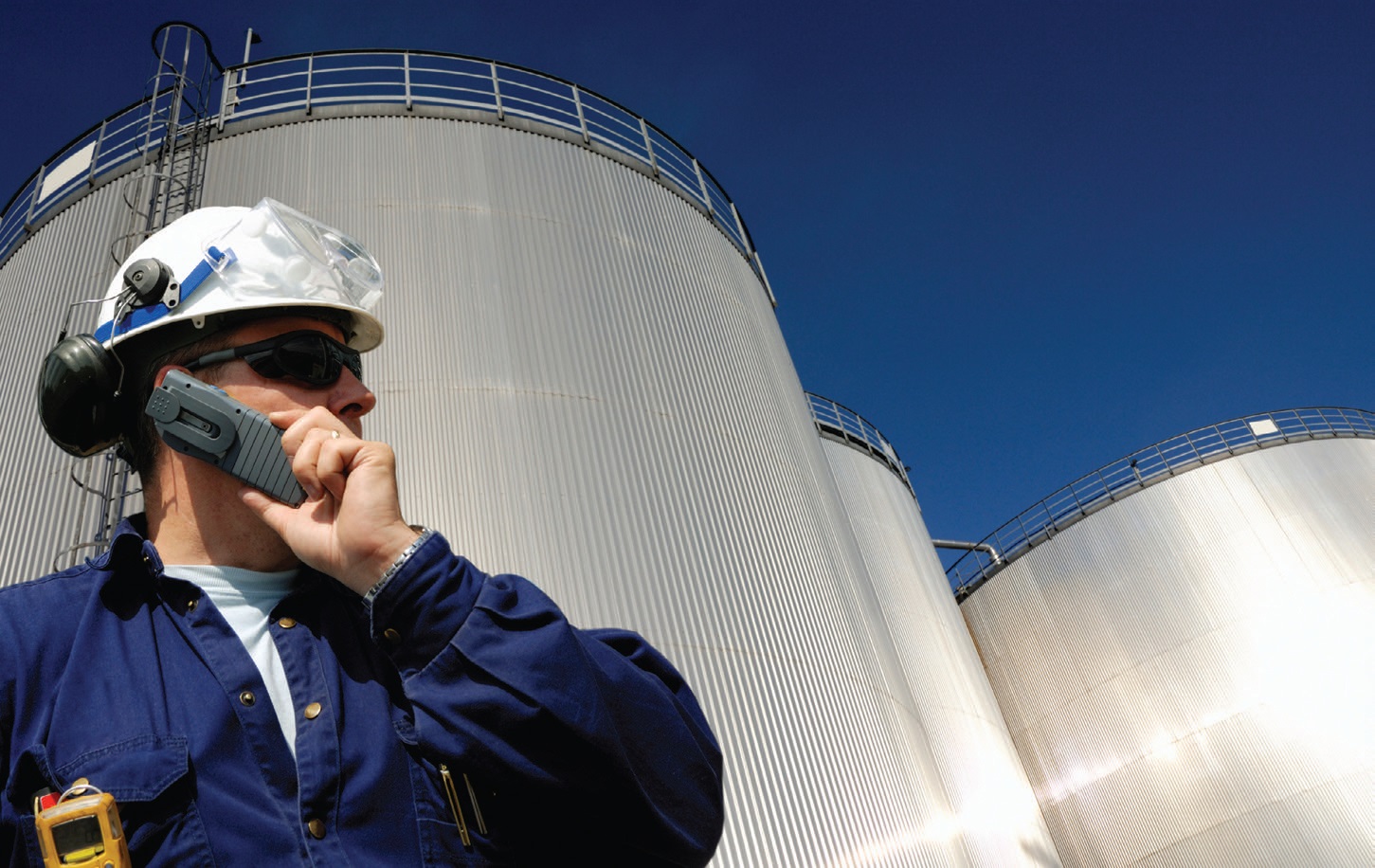
KEY CONCEPTS
•
Analysis alarms can be characterized as statistical, absolute and percentage type measurement.
•
Statistically derived wear debris limits should be developed around individual machine types and designs.
•
Providing a new oil baseline for each product type is absolutely essential.
•
The analyst needs as much detail as can be reasonably provided by the machine owner to make good decisions.
This is intended to introduce and discuss key principles and parameters that management should consider when implementing a oil sampling and analysis plan.
In April we discussed how oil analysis is the feedback loop telling the practitioner whether the lubrication activities are delivering the results expected. Oil analysis should provide information about the state of the lubricant condition, the cleanliness of the sump and the condition of the machine. A variety of tests are used to deliver this type of information. The tests provide insight into machine operating states by focusing on lubricant health, sump/lubricant contamination conditions and changing machine health.
The progressions to failure of most lubricated components are predictable. Lost lubricant health and excessively high contaminant levels lead to two- and three-body abrasive wear, fatigue wear and adhesive wear. The initial wear rate is imperceptibly low, occurring without recognition. Gradually each wear mode causes increased component surface degradation, accelerating the wear mode to a recognizable state, which is, regrettably, well past an opportunity to make productive corrections.
Each of these wear modes provides distinct signatures and can be identified early in the wear-development profile if the sampling and analysis program is conducted with consistency and purpose.
In May and July we mentioned the many different standardized tests that can identify changes in each of these three areas. Some tests provide insight into more than one area of concern. The tests are grouped into primary and secondary test slates. The primary test performs the function of a compass that points the user in the general direction, where the secondary test could be likened to a GPS position finder that tells the user where they are relative to the desired positions. With the additional information, the user then can make decisions that will suit the long-term interests of the organization.
The test slates are assembled based on their utility for a set of machine operating conditions. It is important to select those tests that provide maximum coverage for all three areas of interest for the available expense dollar. Results from primary test slates are then used to trigger the use of more detailed and often more expensive secondary tests.
For each of these areas, there are multiple approaches to setting alarms, which is the central consideration for this article. The three general types of alarms are:
1.
Statistical alarm (used with wear debris analysis)
2.
Absolute alarm (used for a combination of both oil health and sump condition-control analysis)
3.
Percentage-based alarm (used to identify lubricant health and sump contamination changes).
Absolute and percentage-based alarms are sometimes referred to as aging and target-based alarms. Each of these alarm types are addressed in this article.
To get the best information out of wear debris data, one must create alarms based on statistical analysis of data for a specific machine.
STATISTICAL ALARMS
Routine wear debris (metals) analysis produces a data point indicating the number of parts per million of a variety of metals found in the sample. The metals of primary concern are those metals that are known to be used in component construction, including iron, copper, tin, lead, nickel, chromium and aluminum. There are other metals also noted but not common to machine component construction, including silver, titanium, vanadium and molybdenum.
There are also metals (calcium, potassium, boron, sodium, silicon, zinc, phosphorous and antimony) that are typically associated with the additives package in use and the contaminant types likely to be found in the production environment. Flagging the correct metals is every bit as important as flagging the metals at the appropriate level. The statistical alarm methods for wear debris pertain to the first group. It is essential that the reliability engineer understand the specific types of metals in use in every machine under analysis. Component composition information is available from the OEM but may require some digging to uncover.
There are some useful default wear metal limits provided by industry associations and OEMs. For example, the American Gear Manufacturers Association has guidelines for gear wear limits. Many over-the-road (OTR) engine manufacturers have guidelines for wear as do several industrial equipment and component manufacturers. The concern with OEM data is that this information is based on an average of data from machines that were likely not running in your plant’s environment.
To get the best information from wear debris data, one must create alarms based on statistical analysis of data for a specific machine. Ideally, the more data gathered, the more accurate the alarm set. However, in oil analysis, gathering high sample counts for a specific machine could take years depending on the sample interval. For this reason, many oil analysis professionals have chosen to group initial alarm sets by either component type and/or divide machines into make and model and build alarm sets around machine types.
When calculating for appropriate wear debris alarms, a simple standard deviation formula is used. When using standard deviation to create alarms, the initial idea is to be able to focus on the top 5% of equipment with problems.
When gathering the data for statistical analysis, ensure that the data is representative of the machine that is to be governed by the alarm set. For example, sample data from a Falk 1040FZ gearbox should not be included in the data set used to set alarms for a Chemineer 6-HTN-10 gearbox. However, data from both of the above examples could be used if the goal is to have a generic gearbox alarm set. There are some instances when using a generic gearbox alarm set may be the best approach, although continuous improvement practices mentality suggests that wear debris alarms should be reviewed at least annually in order to help fine tune the alarms to an eventual component-specific basis.
Periodically the data should be reviewed for the presence of outliers and adjusted accordingly. Outliers include data that are unusually low or unusually high and are obviously not part of a normal data scheme. For instance, if a Falk 1040FZ gearbox has a historical iron value in parts per million (PPM) running in the mid- to upper-teens, then experiences a catastrophic condition whereby the last sample drawn is well into three digits, including this last value for statistical alarm sets will produce an artificially high alarm point. Too many instances of outliers in the data set can contribute to misleading early alarm (plus one, plus two standard deviations) and missed opportunities.
Another important consideration for maintaining highly representative statistical alarms is the use of rate-of-change limits. This requires careful logging of equipment runtime where the actual wear generation is calculated based on equipment runtime. Wear conditions are then flagged based on generation per run time unit (i.e., hour, mile, cycle). A fully devised rate-of-change limit requires tracking all oil additions, losses and filtration time and then factoring any dilution of the wear debris into the runtime unit. While the latter methods can be used to develop a very precise level of wear debris alarms, it may not be feasible to put these practices in place for every machine sump in the plant receiving analysis.
In most locations, applying simple standard deviation alarms to the component model level is sufficient in achieving 95% of reliability objectives related to lubrication.
Figure 1. Standard deviation formula used to calculate values for alarm intervals (plus one, plus two, plus three standard deviations).
Figure 2. Standard deviation confidence values.
AGING ALARMS
Lubricant health alarms are sometimes called aging alarms or aging limits. While, theoretically speaking, aging limits can be applied to wear debris in addition to fluid properties, aging limits related to machine condition are beyond the scope of this article.
Some common test parameters that indicate lubricant health include:
•
Viscosity
•
AN/BN
•
Additive levels (zinc, phosphorus, calcium, magnesium, boron, barium, antimony)
•
Dielectric constant
•
RPVOT.
When applying alarms to these parameters, it is vital to obtain the property values of the new oil. For example, a general rule for viscosity alarms for industrial oils is a first-level (alarm) for any change of +/-5% from the new oil baseline, and a second-level (alert) for any change of +/-10% from the baseline. The second alarm may be a condemning limit based on machine criticality (which is predicated on risk to safety, productivity and cost concerns as discussed in the January 2008 TLT article). Obviously, without knowledge of the new oil property, it is impossible to correctly assign the proper alarm point.
For example, consider the use of an ISO 220 gear oil. Table 1 indicates how alarms would be different based on the assignment of these alarms. The first column indicates the alarm states if the ISO grade profile is used as the baseline. The second column suggests what the alarm states would be if an actual new ISO 220 gear oil, as provided by the supplier, has an actual baseline viscosity of 200 cSt. This is still within grade for an ISO 220 gear oil and considered acceptable for use, but is nonetheless at the lower limit of acceptability for a new oil.
Table 1. Alarm Value Changes Based on Differences in the Starting Points.
If the alarm set is used for a generic 220 baseline, it is possible to miss a potential failure mode such as increasing oxidation and sludge buildup or the topping of the sump with an incorrect product.
As mentioned earlier, the use of a correct baseline sample is vital. As seen in Figure 3 for an ISO 320 analysis report, there was a noteworthy change in the oxidation, AN and viscosity properties. Without a review and update of the baseline, this sample would have triggered a severe condition.
Figure 3. Fluid properties analysis ensuring proper baselining.
The following values represent general guidelines for aging alarms:
Acid number. 1.0 increase over baseline sample for most industrial oils (there are exceptions to this rule for lubricants with high starting points).
Base number. 50% decrease from baseline sample.
Additives. 25% change from baseline sample.
Dielectric constant. 0.1 increase over baseline sample.
RPVOT. 25% of new oil value.
TARGET ALARMS
Contamination alarms are commonly referred to as target alarms. These are provided as a means to extend machine and lubricant lifecycles. Some of the most common parameters to receive target alarms include:
•
Solids (wear and dirt) via Particle Count (ISO Cleanliness Code)
•
Water
•
Glycol.
In terms of particle counting, an increase in just a single ISO cleanliness level can mean as much as a 4x increase in contamination. Using the same component type mentioned above, Figure 4 shows the life extension potential by simply improving cleanliness on an industrial gearbox.
Figure 4. Gearbox lifecycle improvement targets from improvements in ISO Cleanliness (1).
Several factors determine the ideal target values for various components. While many OEMs have established minimum guidelines, OEM recommendations may not be consistent with an individual plant’s reliability objectives.
Following are a few questions that should be asked when determining component dryness and cleanliness targets that should align with the concerns over general reliability that were addressed in the January 2008 TLT article, including:
1.
How important is the machine to fulfilling daily production requirements?
2.
Is there a safety or environmental concern for/from machine failure?
3.
What is the production opportunity cost from machine failure?
4.
How sensitive is this component to water/particle contamination?
5.
How quickly does the oil separate from water?
6.
What are the running cost, repair and downtime charges due to water/particles?
Some common water limits based on component type include:
•
Rotary screw and centrifugal compressors—500 ppm.
•
Turbine and components using circulating oils—200 ppm.
•
Hydraulic systems—100 ppm.
•
EHC systems—100 ppm.
•
cIndustrial gearboxes—400 ppm.
The examples given in this article are simply general guidelines. As mentioned in each section, customization can and should take place based on actual reliability objectives. Properly setting alarms for your equipment can mean the difference in finding a potential problem before damage is initiated or finding a problem when failure is already imminent.
SUMMARY
Following selection of a test slate, the reliability manager must apply an alarm structure for each test. There are three common types of alarm mechanisms, including statistical, percentage and absolute measurements. The background data points used to create statistical alarm sets should be limited by machine type, make, model and operating state, if possible. General statistical alarms are commonly applied to machine wear debris. The data requires periodic vetting of outliers in order to remain viable over time. Absolute and percentage-based alarms are routinely applied to lubricant health and contamination control tests.
Lubricant health alarms (of either type) cannot be set without a predefined baseline—a fingerprint of the lubricant—against which either percentage changes or differential measurements are provided. The baseline should be frequently renewed for each product type in use.
REFERENCE
M. Moon,
Gearsolutions.com, June 2009, showing: J. Fitch.
Practicing Oil Analysis Magazine. Sept. 2005.
Mike Johnson, CLS, CMRP, MLT, is the principal consultant for Advanced Machine Reliability Resources, in Franklin, Tenn. You can reach him at mike.johnson@precisionlubrication.com.
Matt Spurlock, CMRP, MLA II, MLT I, LLA I, is the machine lubricant subject matter expert at Allied Reliability, Inc., in Indianapolis, Ind. You reach him at spurlockm@alliedreliability.com.