Design of experiment reduces development time for higher-performing metal-cutting fluids
David Slinkman and Yixing (Philip) Zhao | TLT Metalworking January 2018
The software and process helped create a new product with half the number of formulations typically needed.
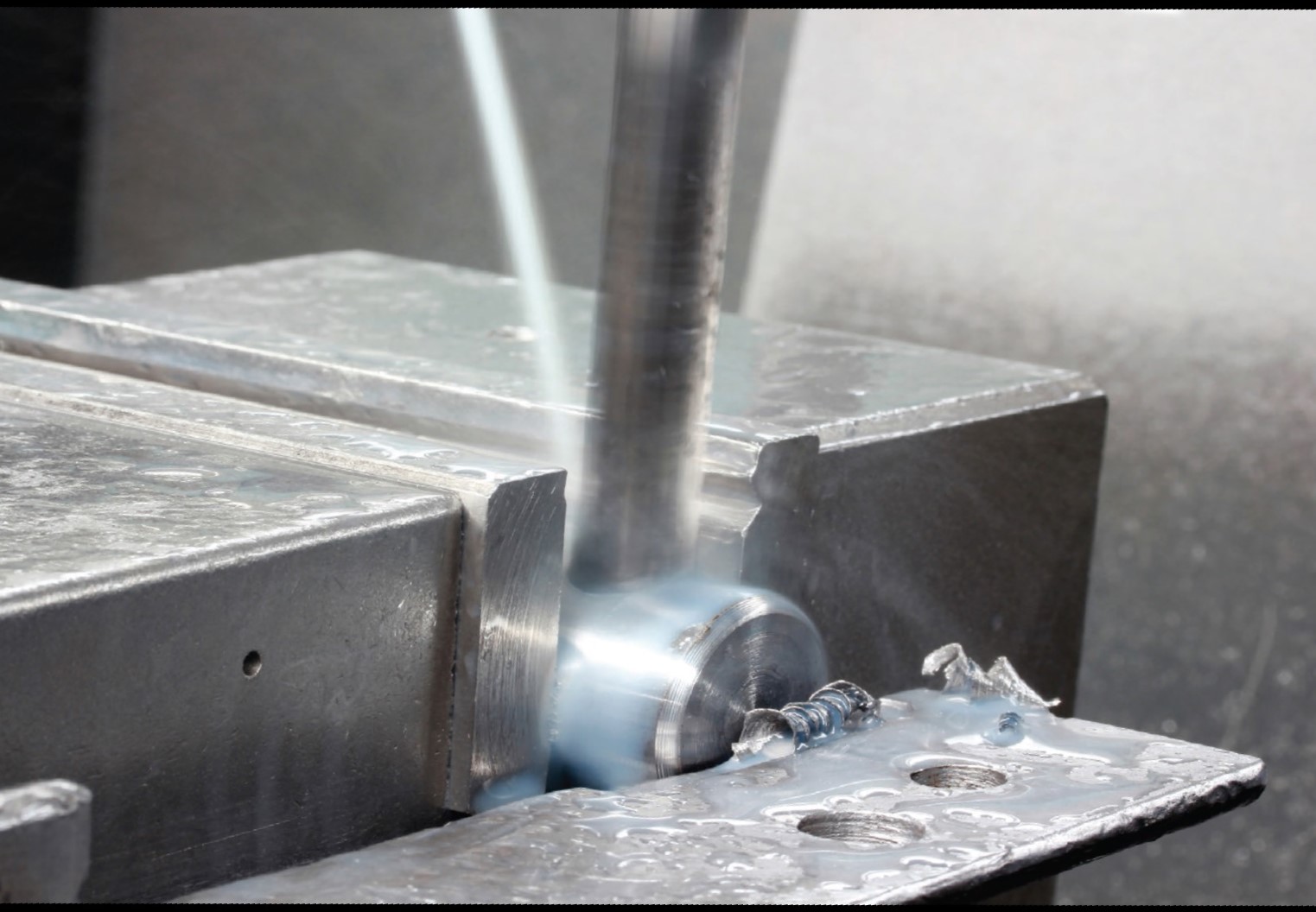
© Can Stock Photo / SafakOguz
KEY CONCEPTS
•
The large number of interactive ingredients makes developing MWFs a complex process.
•
Design of experiment (DOE) methodology recently helped chemists develop an MWF using half the number of formulations typically necessary.
•
The DOE software accurately projected that the emulsion stability of the optimized formulation would be substantially better than the current product.
DEVELOPMENT OF METALWORKING FLUIDS (MWFs) and other specialty chemical products is complicated by the large number of ingredients with interactive effects on the properties of the finished product. In the past, chemists typically made 400 or more formulations from the inception to the completion of a project while testing the effects of each ingredient individually.
More recently, Houghton has moved to using design of experiments (DOE) to simultaneously assess the effects of many ingredients, including any interactions between ingredients. Recently chemists used this approach to develop a new metal-cutting fluid with superior emulsion stability while making fewer than 200 formulations.
ROLE OF MWFs
MWFs play an important role in machining metal parts by keeping the workpiece at a stable temperature so close tolerances can be held. They also lubricate the cutting tool to increase its life, prevent rust on workpieces and cutters and help improve the quality of the workpiece by continuously removing metal fines, chips and swarf from the cutting tool and surface of the workpiece.
Achieving all the properties required for an MWF typically requires a carefully designed formulation with approximately 20 different ingredients, including lubricity additives, emulsifying agents, wetting agents, detergents, anti-foaming agents, etc. Each of these ingredients has complicated effects on the properties of the final product. Frequently ingredients interact with each other in difficult-to-predict ways.
Many MWFs are used as emulsions, a system of two materials that do not mix with each other in which one material is suspended or dispersed throughout the other material in separate droplets. Most commonly, tiny oil droplets are surrounded by water. An emulsifier molecule consists of a water-loving hydrophilic head and an oil-loving lipophilic (or hydrophobic) tail. The balance between the hydrophilic portion and the lipophilic portion of the emulsifier is called the Hydrophile-Lipophile Balance (HLB). An emulsifier that is mostly lipophilic is assigned a lower HLB value (below 9.0), and one that is mostly hydrophilic is assigned a higher HLB value (above 11.0). Those in the range of 9-11 HLB values are intermediate.
PREVIOUS FORMULATION METHODS
In the past, chemists prepared many formulations and measured their properties to assess the effects of the different additives. This approach is time-consuming but effective in determining single-factor effects. But it is difficult, if not impossible, to understand multiple-factor interactions using the one-factor-at-a-time method. The inability to understand these interactions often led to surprises that required additional work—just when the chemists thought they were close to achieving their product performance objectives. Furthermore, not taking advantage of multiple factor interactions sometimes led to performance that was good enough but lower than optimal values.
A recent project developed a replacement for an existing emulsifiable oil. It is designed to form a stable emulsion when mixed with water. The goal for the new product was to offer enhanced emulsion stability and also reduce foaming while maintaining good blooming characteristics. (Blooming refers to the ease and speed with which the fluid concentrate mixes with water.) Concentrate stability is important because it allows a product to be stored for longer periods of time. Chemists also wanted to limit the amount of foaming in the new product because foam may obscure the operator’s vision of the workpiece and create housekeeping problems.
RUNNING THE EXPERIMENT
Chemists used DOE software on this project that was easy to use and required very little statistical background. The software made it possible to efficiently set up a DOE matrix for formulation development. Data analysis such as Analysis of Variance (ANOVA) and graphing was enabled with the software.
In this case, chemists used the software to set up a factorial design to study the correlations and interactions of selected additives. They wanted to investigate the effects of changing the concentration of three different emulsifiers with medium (Emulsifier A), low (Emulsifier B) and high (Emulsifier C) HLB numbers. They selected three levels of concentration for each emulsifier. Factors and concentrations were populated and the software generated a test matrix with nine runs (
see Table 1). The chemists then prepared and ran a series of evaluations for each formulation.
Table 1. DOE Sample Matrix
In the emulsion blooming test (
see Figure 1), the concentrate is injected into water and chemists record the emulsion travel distance of each sample. The emulsion stability was evaluated by Turbiscan where the sample is scanned—in this case, from bottom to top with an 850-nm monochrome light beam while collecting backscattering readings. They are dependent on oil droplet size and concentration. Any destabilization in a given sample changes the backscattering intensity. Turbiscan profiles are collected over 24 hours. The backscattering value at a given height is charted as a function of time to create a kinetic profile at a particular tube position (
see Figure 2). A sample with a constant backscattering value possesses the highest possible stability. Chemists also measured foaming performance via proprietary evaluations.
Figure 1. Emulsion blooming test results.
Figure 2. Emulsion stability test results.
CORRELATIONS OF ADDITIVE EFFECTS
The chemists entered the measurements of the nine runs into the DOE software, which generated a variety of statistics. The software’s statistical analysis showed:
•
Emulsifier A increases blooming speed, B has a negative effect and C is not significant (
see Figure 3).
•
The AC emulsifier interaction improves the blooming when the concentration of C is low. However, the BC emulsifier interaction decreases blooming when the level of C is low, whereas the blooming will be improved at higher concentration of C (
see Figure 4).
•
Emulsion stability testing showed that A and B reduce emulsion stability, and C increases emulsion stability (
see Figure 5).
Figure 3. Output from design expert showing main additive effects on blooming.
Figure 4. Output from design expert showing interaction effects on blooming.
Figure 5. Output from design expert showing main additive effects on emulsion stability.
Obtaining this information in only nine runs is a major success since in the past it could take hundreds of runs to obtain these basic trends without knowing the quantitative effects of the interactions that were provided by DOE.
OPTIMIZATION RESULTS
The DOE software then mapped out the formulation space and determined optimized values for each of the factors based on achieving at least the performance of the old product in blooming and foaming, along with the highest possible value for emulsion stability (
see Figure 5). The software allows us to set up the ranges of emulsifiers (factors) and expected properties of new formula (responses) in the optimization step (
see Table 2).
Table 2. Parameter Selection for Formula Optimization
The chemists prepared a sample of this formulation and ran the same tests on it. The DOE software projected that the emulsion stability of the optimized formulation would be substantially better than the current product—a 19% change in backscattering at 24 hours for the current product versus a 6% backscattering change for the optimized formula (
see Figure 6). The test results showed that foaming and blooming were both within the range projected by the experiment while the emulsion stability was slightly better than the projected range (
see Table 3). A new product was introduced based on the optimized formula, and it has been a success in the market. All in all, this application of DOE saved significant time, making it possible to provide customers with improved technology in an expedient manner.
Figure 6. Improved emulsion stability of optimal formula (green) versus previous product (red).
Table 3. DOE Optimal Formula and Comparison
All stats were compiled using Design-Expert® software from Stat-Ease, Inc. (
www.statease.com).
For more information, contact Houghton International Inc. at www.houghtonintl.com.
Dr. David Slinkman is senior vice president, Global Research and Technology, for Houghton International in Valley Forge, Pa. You can reach him at dave.slinkman@houghtonintl.com.
Dr. Yixing (Philip) Zhao is Houghton’s senior research scientist-innovation team leader. You can contact him at philip.zhao@houghtonintl.com.