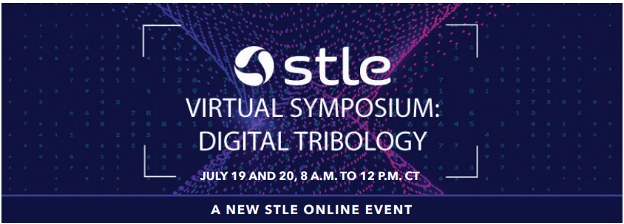
Schedule-At-A-Glance
(As of July 6, 2023) – Schedule subject to change.
All times are in US Central Time (CT)
Register today for the Virtual Symposium
Registration Pricing
$99 - STLE Members
$149 - Non-members
$25 - Students
*Registration include access to the live technical presentations on both days and on-demand recordings.
Day 1: Wednesday, July 19, 2023
Theme: Data
8:00 am – 8:10 am
Welcome
8:10 am – 9:00 am
Polymer Informatics
Speaker: Rami Ramprasad, Georgia Institute of Technology (USA)
The Materials Genome Initiative (MGI) has heralded a sea change in the philosophy of materials design. In an increasing number of applications, the successful deployment of novel materials has benefited from the use of computational, experimental and informatics methodologies. Here, we describe the role played by computational and experimental data generation and capture, polymer fingerprinting, machine-learning based property prediction models, algorithms for designing polymers meeting target property requirements, and above all, how prior physics knowledge may be incorporated with polymer informatics workflows. These efforts have culminated in the creation of an online Polymer Informatics platform (https://www.polymergenome.org) to guide ongoing and future polymer discovery and design [1-3]. Challenges that remain will be examined, and systematic steps that may be taken to extend the applicability of such informatics efforts to a wide range of technological domains will be discussed. These include strategies to deal with the data bottleneck, new methods to represent polymer morphology and processing conditions, and the applicability of emerging AI algorithms for materials design.
Speaker Profile
Prof. Ramprasad is presently the Michael E. Tennenbaum Family Chair and Georgia Research Alliance Eminent Scholar in the School of Materials Science & Engineering at the Georgia Institute of Technology. His area of expertise is the development and application of computational and machine learning tools to accelerate materials discovery, as applicable to energy production, storage and utilization. Prof. Ramprasad received his B. Tech. in Metallurgical Engineering at the Indian Institute of Technology, Madras, India, an M.S. degree in Materials Science & Engineering at the Washington State University, and a Ph.D. degree also in Materials Science & Engineering at the University of Illinois, Urbana-Champaign .Prof. Ramprasad is a Fellow of the Materials Research Society, a Fellow of the American Physical Society, an elected member of the Connecticut Academy of Science and Engineering, and the recipient of the Alexander von Humboldt Fellowship and the Max Planck Society Fellowship for Distinguished Scientists. He has authored or co-authored over 225 peer-reviewed journal articles, 8 book chapters and 8 patents, and has delivered over 300 invited talks at Universities and Conferences worldwide. He is a member of the Editorial Advisory Boards of npj Computational Materials, ACS Materials Letters and Journal of Physical Chemistry A/B/C, and is the Chair of the inaugural 2022 Gordon Research Conference on Computational Materials Science and Engineering.
9:00 am – 9:50 am
Two-Tier Machine Learning Acceleration of Molecular Dynamics with Enhanced Sampling
Speaker: Lixin Sun, Microsoft Research Cambridge
Computing accurate reaction rates is a central challenge in computational materials, chemistry and biology. This is due to the high cost associated with free energy estimation using unbiased molecular dynamics. In this talk, we will review a two-tier framework designed to accelerate free energy estimation by utilizing machine learning. Our discussion will also include various data-driven algorithms for interatomic potentials and collective variable discovery, as well as their applications to complex reaction rate calculation problems. Future challenges and potential method developments are also addressed.
Speaker Profile
Lixin Sun is a senior researcher at Microsoft Research, Cambridge, UK, working in the AI4Science team. Her research focuses on accelerating materials R&D for sustainable technologies with machine learning and automatic workflows. Her specific area of interest lies in accelerating atomistic modeling for long-time-scale and large-length-scale phenomena. Prior to joining Microsoft, she has received her PhD from MIT and worked as a postdoctoral researcher at Harvard. Her previous research includes developing machine learning method and conducting case studies for catalysis and energy materials.
9:50 am – 10:00 am
Break
10:00 am – 10:50 am
Machine Learning Interatomic Potentials: Past, Present, Future
Speaker: Shyue Ping Ong, University of California, San Diego (USA)
Machine learning interatomic potentials (MLIPs) enable the accurate simulation of materials at larger sizes and time scales, and play increasingly important roles in the computational understanding and design of materials. This talk will discuss the past, present and future of MLIPs, with a focus on the different architectures that have been developed over the years and their myriad technological applications. We will outline the major challenges that remain, particularly with respect to the generation of accurate training data, and potential areas for further development.
Speaker Profile
Shyue Ping Ong is a Professor of NanoEngineering at the University of California, San Diego. He obtained his PhD from the Massachusetts Institute of Technology in 2011. He leads the Materials Virtual Lab at UCSD, a dynamic group of materials scientists focusing on the interdisciplinary application of materials science, computer science, and data science to accelerate materials design. He is one of the founding developers of the Materials Project, a DOE-funded initiative to make the computed properties of all known materials publicly available for materials innovation. He is also the founder of Python Materials Genomics (pymatgen), an open-source materials analysis library that is used by hundreds of thousands of users worldwide. Dr Ong is a recipient of the US Department of Energy Early Career Research Program and the Office of Naval Research Young Investigator Program awards and a Clarivate Highly Cited Researcher in 2021 and 2022.
10:50 am – 11:40 am
Molecular Dynamics Simulations and Machine Learning for Predicting Lubricant Properties
Speaker: Ashlie Martini, University of California, Merced (USA)
Relationships between base oil and additive molecular features and lubricant properties are complex and still not fully understood. Here we used molecular dynamics simulations combined with machine learning to identify structure-property-function relationships for lubricants. First, we simulated blends of synthetic base oil with polymeric lubricant additives to predict viscosity index, thickening efficiency, and traction coefficient. Results were validated by direct comparison to experimental data. The molecular origins of observed trends were investigated using molecular properties readily calculated from the simulations to develop simple empirical models that predict viscosity index, thickening efficiency, and traction coefficient. Next, we developed a suite of open-source post-processing routines for calculating more than 2,000 3D molecular descriptors from atomic trajectories-generated molecular dynamics simulations. Lastly, we demonstrated the utility of the new post-processing tools for predicting the temperature-dependent density and viscosity of hydrocarbon base oils.
Speaker Profile
Ashlie Martini is professor and chair of the Department of Mechanical Engineering at the University of California, Merced. She has been working in the field of tribology for the past 20 years with research reported in 200+ published papers and she is a Fellow of STLE and ASME. She also recently published a textbook titled “Introduction to Tribology for Engineers.” Her contributions to research, as well as teaching and service to the profession, have been recognized by multiple prestigious awards.
11:40 am – 12:00 pm
Panel Discussion
Day 2: Thursday, July 20, 2023
Theme: Tribology
8:00 am – 8:10 am
Welcome
8:10 am – 9:00 am
Data Science Approach in Japanese Tribology
Speaker: Hitoshi Washizu, University of Hyogo (Japan)
From 2020 to 2023, Japanese Society of Tribologists (JAST) started a study group of application of AI to Tribology. In this talk, the presenter will report some of the topic discussed there. The early case was done in 1990s to determine the relation between wear and the debris. Recently, data science approach is used in many fields. Surface texturing of mechanical joint is optimized by AI. Data science is used for remote monitoring of lubricant condition in construction machinery and earned additional customers. In our laboratory, we used material informatics to find new lubricant molecules. In material science, new approach using neural network potential for molecular dynamics is applied to understand the dynamics and chemical reaction of additives on the surface. Since tribology phenomena is complex from material to machine itself, data science approach is useful when the tribologists set the problem carefully.
Speaker Profile
Hitoshi Washizu received his Ph.D. from University of Tokyo in physical chemistry (molecular simulation of polymer solution). His main research field is dynamics of molecules on surfaces, especially in tribology. Based on his 14-year career in automotive laboratory, work in MEXT next-generation supercomputer project, Element strategy project, NEDO wind turbine project, he is a specialist in supercomputing and collaboration research with industries. He is also Research Center Professor at the Elements Strategy Initiative for Catalysts and Batteries (ESICB), Kyoto University.
9:00 am – 9:50 am
Tribology in Multifields
Speaker: Q. Jane Wang, Northwestern University (USA)
A tribology interface can be a multifield energy exchanger facing interactions of mechanical, thermal, chemical, and electromagnetic forces. We recently worked on the theories and methods of several tribological issues in mechano-electromagnetic fields. This presentation discusses (1.) a general theory of multifield contact problems with an application on the analysis of thermomechanical-electrical sliding wear of materials with rough surfaces, (2.) cross-field analogy of multifield systems and a mechanics method for multifield problems with an application on the simulation of ion transport through a porous electrolyte in an all solid-state battery, and (3.) multifield lubrication problems and the development of a generalized, multifield Reynolds equation for EV applications.
Speaker Profile
Q. Jane Wang is a professor of mechanical engineering at Northwestern University. She has devoted her research to advancing the field of tribology—the study of friction on surfaces. Her research group studies tribological interfaces from integrated theories for contact and interfacial mechanics, numerical simulations, model-based designs of machine elements and surfaces, failure-prevention methods, and novel lubrication technologies, working with industries toward future technologies for energy efficient, highly reliable machinery.
As executive director of Northwestern’s Center for Surface Engineering and Tribology (CSET), she leads the center’s efforts to address critical problems related to surface failure that affects key components in advanced engines, manufacturing equipment, bearings, batteries, and biomechanical systems. CSET’s research has supported advances in industries such as aerospace, transportation, electronic and data processing and biomedical engineering. She has published nearly 300 journal articles and edited four major texts covering topics related to tribology. In 2013, she co-edited “Encyclopedia of Tribology” with STLE member Yip-Wah Chung, professor of materials science and engineering at Northwestern. The six-volume publication marked the first major reference that comprehensively combines the science, engineering, and technological aspects of tribology within a single work.
9:50 am – 10:00 am
Break
10:00 am – 10:50 am
Computational Tools and their Application to Study the Tribological Properties
Speaker: Maria Clelia Righi, University of Bologna (Italy)
This presentation will describe the development of computational tools and their application to study the tribological properties of solid interfaces and design lubricant additives. (1.) We developed a robust, high-throughput workflow able to automatically optimize the structure of solid-solid interfaces and calculate their adhesion energies from first principles. We apply it to screen hundred metallic heterostructures relevant for technological applications. This allows us to populate a database of accurate values, which can be used as input parameters for macroscopic models. Moreover, it allows us to benchmark commonly used empirical relations that link adhesion energies to the surface energies of its constituent, and to improve their predictiveness by employing a machine-learning derived function. (2.) We developed a software for smart sampling the configurations used to train neural network potentials through DeepMD. We will discuss the problems encountered in simulating tribological interfaces and discuss preliminary results in reproducing ab initio molecular dynamics simulations of carbon-based molecules at sliding interfaces.
Speaker Profile
Marie Clelia Righi is a professor in the Department of Physics and Astronomy at the University of Bologna. Her research focuses on the development and application of computational methods to understand and predict the behavior of materials from first principles, particularly of surface and interface phenomena. She adopted pioneering computational approaches in tribology and applied them for understanding chemical reactions activated by mechanical stresses and designing materials to reduce friction. In 2019, she received an ERC consolidator grant for the project “Advancing solid interfaces and lubricants by first principles material design.” She is a visiting professor at the Imperial College London, UK, and collaborates with different multinational companies and international experimental labs. She is part of the editorial boards of Coatings, Lubricants, Lubrication Science, and Scientific Reports.
10:50 am – 11:40 am
Explainable Al via Semantic Information Pursuit
Speaker: René Vidal, University of Pennsylvania (USA)
There is a significant interest in developing Machine Learning (ML) algorithms whose final predictions can be explained in terms understandable to a human. This has motivated a number of approaches that seek to provide explanations for existing ML algorithms in a post-hoc manner. However, many of these approaches have been widely criticized for a variety of reasons and no clear methodology exists in the field for developing ML algorithms whose predictions are readily understandable by humans. To address this challenge, a method for constructing high-performance ML algorithms which are “explainable by design” was developed. This method makes its prediction by asking a sequence of domain- and task-specific “Yes/No” queries about the data (akin to the game “20 questions”), each having a clear interpretation to the end-user. We then minimize the expected number of queries needed for accurate prediction on any given input. This allows for human interpretable understanding of the prediction process by construction, as the questions which form the basis for the prediction are specified by the user as interpretable concepts about the data. Experiments on language, vision and biomedical tasks demonstrate the efficacy of our approach and its superiority over post-hoc explanations.
Speaker Profile
René Vidal is the Penn Integrates Knowledge and Rachleff University Professor of Electrical and Systems Engineering & Radiology and the Director of the Center for Innovation in Data Engineering and Science (IDEAS) at the University of Pennsylvania. He also directs the NSF-Simons Collaboration on the Mathematical Foundations of Deep Learning and the NSF TRIPODS Institute on the Foundations of Graph and Deep Learning. He is also an Amazon Scholar, Affiliated Chief Scientist at NORCE, and Associate Editor-in-Chief of TPAMI. His current research focuses on the foundations of deep learning and trustworthy AI and its applications in computer vision and biomedical data science. He is an ACM Fellow, AIMBE Fellow, IEEE Fellow, IAPR Fellow and Sloan Fellow, and has received numerous awards for his work, including the IEEE Edward J. McCluskey Technical Achievement Award, D’Alembert Faculty Award, J.K. Aggarwal Prize, ONR Young Investigator Award, NSF CAREER Award, as well as best paper awards in machine learning, computer vision, controls, and medical robotics.
11:40 am – 12:00 pm
Panel Discussion